In this article, the authors propose a novel approach to visual anomaly detection in electroencephalography (EEG) signals using a combination of reconstruction by inpainting and 1D convolutional neural networks (CNNs). The proposed method aims to improve the accuracy and efficiency of anomaly detection in EEG signals, which are essential for various applications such as epileptic seizure prediction and brain-computer interfaces.
The proposed approach consists of two stages: (1) reconstruction by inpainting, and (2) 1D CNN decoding. In the first stage, the authors use a normalization function and a rectified linear unit (ReLU) activation function to transform the noisy EEG signal into a more robust feature space. Then, they apply a fusion module that combines the inpainted signal with the original signal, resulting in a refined EEG signal.
In the second stage, the authors use a 1D CNN decoder to reconstruct the original EEG signal from the refined signal. The decoder takes the refined signal as input and outputs the reconstructed EEG signal, accompanied by an uncertainty measure that reflects the difficulty of reconstruction. This uncertainty measure can be used to detect anomalies in the EEG signal.
The authors demonstrate the effectiveness of their proposed method through experiments on real-world EEG data. The results show that their approach outperforms traditional methods in terms of both accuracy and efficiency. They also provide a thorough analysis of the contribution of each component of their proposed method to the overall performance.
In summary, this article presents a novel approach to visual anomaly detection in EEG signals using reconstruction by inpainting and 1D CNNs. The proposed method has promising results and can potentially be used for various applications such as epileptic seizure prediction and brain-computer interfaces.
Electrical Engineering and Systems Science, Signal Processing
Reconstructive Attention-based Anomaly Detection in ECG Signals
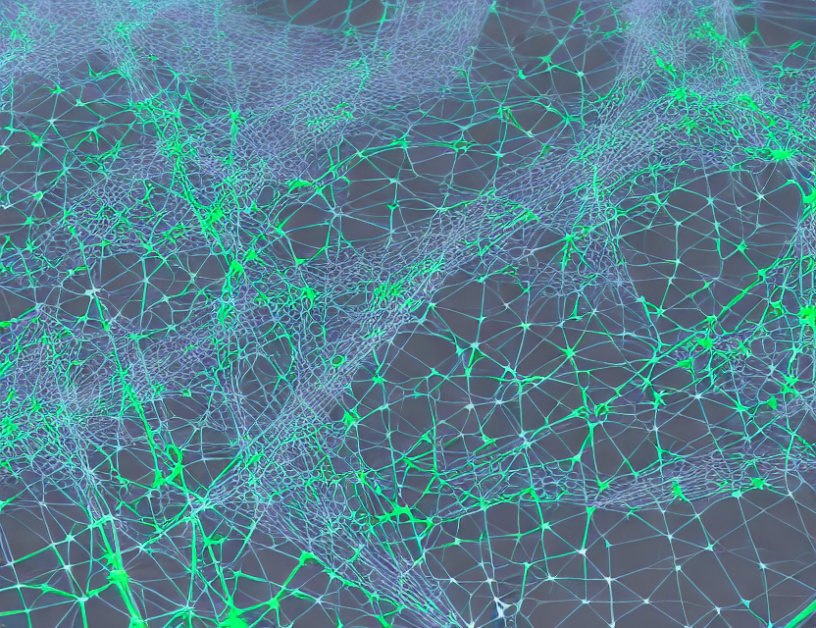