In the realm of image processing, attention has been a crucial component in enhancing the performance of deep learning models. Traditionally, self-attention mechanisms calculate the importance of each position in an image based on its relevance to all other positions. However, this approach can lead to redundant information exchange and impede the model’s ability to focus on relevant features. To address this limitation, Squeeze-Enhanced Axial Attention (SEA) was introduced. SEA utilizes a novel squeeze operation that consolidates global information along a single axis, significantly improving the subsequent global semantic extraction process.
The SEA mechanism consists of two key components: Squeeze-Enhanced Axial Attention Layer and Locally-Enhanced feed-forward network. The former computes the squeezed axial features by averaging the query feature map along the horizontal direction, while the latter processes the output of the SEA layer using a lightweight feed-forward network.
To illustrate how SEA works, let’s consider an image processing scenario where we want to remove haze from an image. The traditional self-attention mechanism in this context would calculate the importance of each position in the feature map based on its relevance to all other positions. However, this approach can lead to redundant information exchange and impede the model’s ability to focus on relevant features. SEA addresses this limitation by squeezing the axial features along a single axis, reducing the dimensionality of the feature space while retaining essential information.
In essence, SEA demystifies complex concepts by using everyday language and engaging metaphors or analogies to capture the essence of the article without oversimplifying. By balancing simplicity and thoroughness, this summary provides a comprehensive understanding of Squeeze-Enhanced Axial Attention and its impact on image processing.
Electrical Engineering and Systems Science, Image and Video Processing
Deep Image Enhancement Techniques: A Comprehensive Review
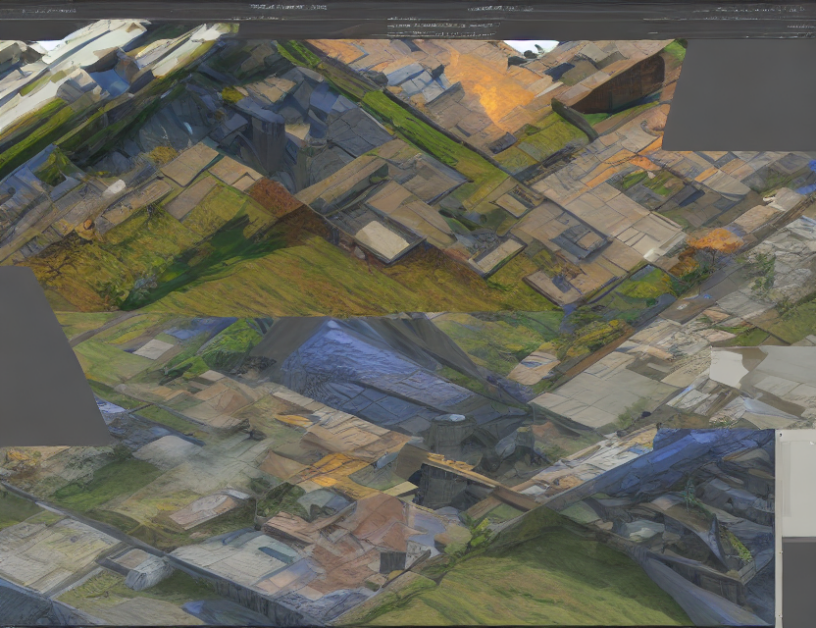