Gliomas are a type of brain tumor that can be difficult to diagnose and treat, especially in low-resource settings where access to advanced imaging tools and skilled personnel is limited. In this article, the authors propose a novel approach called "SAM," which stands for "Segmentation Adaptive Model." SAM is designed to improve the accuracy of glioma segmentation in these settings by adapting to different data qualities and producing accurate segmentations with appropriate guidance.
The authors explain that gliomas are characterized by their heterogeneous and infiltrative nature, which makes them challenging to diagnose and treat. Precise segmentation of the tumor’s sub-regions is crucial for understanding its behavior and guiding personalized treatment strategies. However, achieving precise segmentation remains a challenging task, particularly in low-resource settings where access to advanced imaging tools and skilled personnel is limited.
To address this challenge, the authors propose SAM, an adaptive segmentation model that can improve the accuracy of glioma segmentation in low-resource settings. SAM uses a deep learning approach called convolutional neural networks (CNNs) to learn the patterns in brain images and segment the tumor’s sub-regions accurately. The key innovation of SAM is its ability to adapt to different data qualities, such as image resolution and quality, which are often poor in low-resource settings.
The authors demonstrate the effectiveness of SAM using a dataset from Sub-Saharan Africa, where the imaging quality is generally lower compared to other regions. The results show that SAM can produce accurate segmentations even when the images are of poor quality. The authors also compare the performance of SAM with other state-of-the-art methods and show that it outperforms them in terms of accuracy and robustness.
In summary, this article presents a novel approach called SAM to improve the accuracy of glioma segmentation in low-resource settings. SAM is designed to adapt to different data qualities and produce accurate segmentations with appropriate guidance. The authors demonstrate the effectiveness of SAM using a dataset from Sub-Saharan Africa and show that it outperforms other state-of-the-art methods in terms of accuracy and robustness. This study has important implications for neuro-oncology research and could potentially improve patient outcomes in low-resource settings.
Electrical Engineering and Systems Science, Image and Video Processing
Improving Segmentation Accuracy in Medical Images with SAM and YOLO
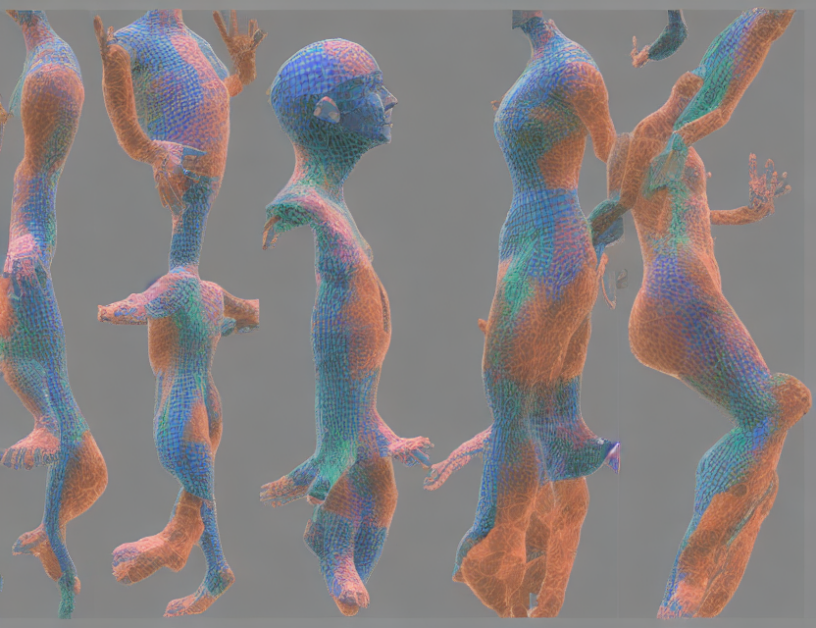