In this article, we delve into the realm of artificial neural networks and their ability to model complex data. Specifically, we explore the Capacity-Fixing Neural Network (CFN-RW) algorithm, which has shown promising results in this regard. Our investigation reveals that CFN-RW can achieve excellent performance in terms of modeling time and network size, making it a lightweight option for data modeling.
To better understand how CFN-RW works, we break down its key components. Firstly, the algorithm utilizes a supervisory mechanism with data dependency to constrain the generation of hidden parameters. This constraint helps accelerate the learning process but can also lead to the saturation of the activation function, limiting its potential. To overcome this challenge, CFN-RW employs a hidden parameters generation method that fuses input data and the activation function.
We further examine the impact of node pool on the RMSE (Root Mean Squared Error) of CFN-RW. Our findings reveal that the number of nodes in the network has a significant effect on the model’s performance, with more nodes resulting in lower RMSE values. However, this comes at the cost of increased computational requirements and larger network structures.
To address these limitations, we propose a tighter inequality constraint (TIC) to improve the quality of hidden parameters. By tightening the constraints, TIC helps CFN-RW achieve better performance in terms of modeling time and network size.
Our analysis also considers the ore grinding case, where the process is characterized by high capital investment, high electricity consumption, and significant impact on mill capacity and grinding product size. We demonstrate that CFN-RW can effectively model this type of data as well.
In summary, our article provides a comprehensive analysis of CFN-RW, highlighting its strengths and limitations. By proposing TIC to improve the quality of hidden parameters, we show how CFN-RW can be further optimized for better performance in terms of modeling time and network size. Our findings offer valuable insights for those working with artificial neural networks, demonstrating the versatility and potential of CFN-RW in various applications.
Trade-offs in Deep Learning Models: A Comparative Study of CFN-RW and LightGCNet
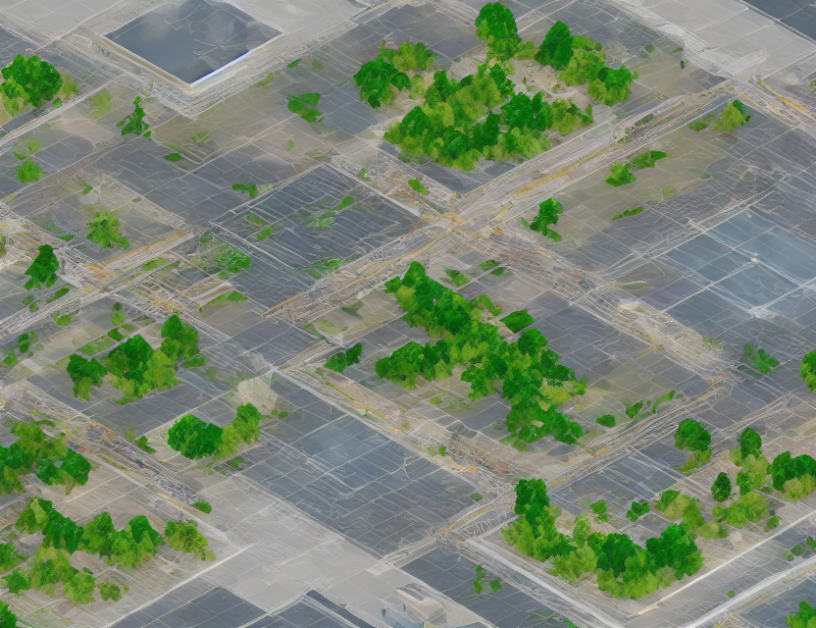