In the realm of data assimilation, ensembles have long been used to approximate complex probability distributions. The Ensemble Kalman Inversion (EKI) framework combines this approach with the Kalman-Bucy filter, allowing for efficient and accurate inversion of inverse problems. This article aims to provide a comprehensive overview of EKI, demystifying its key concepts and highlighting its potential advantages.
At its core, EKI is an iterative method that leverages ensembles to approximate the posterior distribution of a system’s state given observed data. By integrating the Kalman-Bucy filter into this framework, EKI can handle non-linear problems with ease. In essence, it provides a means to "smooth out" the posterior distribution by incorporating the ensemble’s collective knowledge.
To begin, let’s consider an ensemble of possible state paths, each representing a different realization of the system. By iteratively updating these states using observed data, EKI can generate a more accurate estimate of the true state. The Kalman-Bucy filter plays a crucial role in this process, as it enables the estimation of the system’s dynamics and measurements.
One key advantage of EKI is its ability to adaptively increase the size of the ensemble, thereby reducing computational complexity without sacrificing accuracy. This makes it particularly useful for large-scale problems where computation time can be a limiting factor.
Another important aspect of EKI is its inherent ability to incorporate prior knowledge or constraints on the system’s state. By incorporating these constraints into the ensemble, EKI can provide more reliable results by reducing the impact of unrealistic state samples.
So how does it work in practice? Let’s say we have a system with a complex non-linear dynamics and a set of observed data. We begin by generating an initial ensemble of possible state paths and then iteratively update each path using the Kalman-Bucy filter. At each iteration, we compute the Tikhonov regularization term, which helps to stabilize the inversion process and prevent overfitting. Finally, we use the updated ensemble to compute a new set of state samples that better represent the true system state.
While EKI offers many advantages over traditional optimization-based or Bayesian approaches, it is not without its challenges. One potential issue arises when dealing with complex problems that exhibit multiple local optima. In such cases, EKI may struggle to converge to a global minimum, leading to suboptimal solutions.
In conclusion, Ensemble Kalman Inversion represents a powerful tool for solving inverse problems that combines the strengths of ensemble methods and the Kalman-Bucy filter. By adaptively adjusting the size of the ensemble and incorporating prior knowledge or constraints, EKI can provide more accurate and reliable results than traditional approaches. While it may encounter challenges in complex problems, its potential advantages make it a valuable methodology for tackling a wide range of applications.
Mathematics, Numerical Analysis
Ensemble Kalman Methods: A Mean Field Perspective
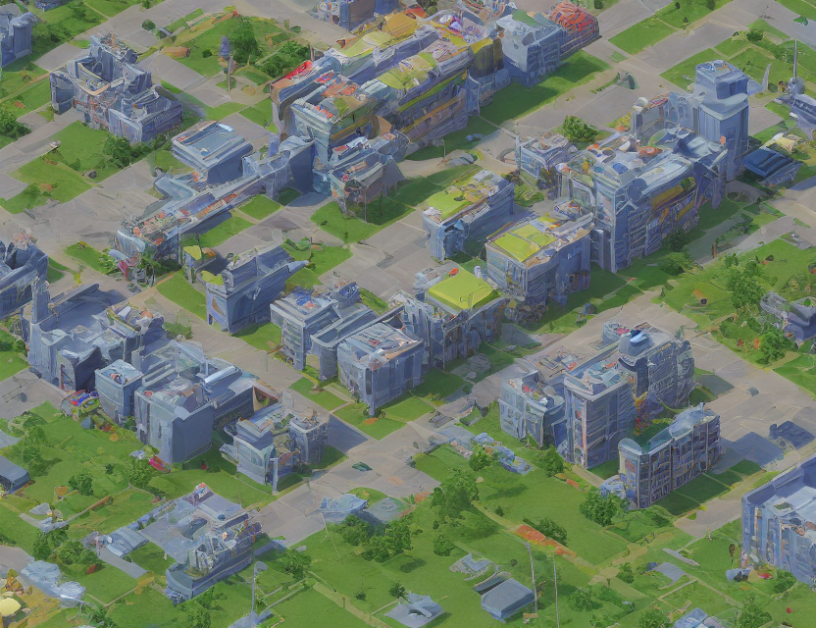