In this article, we propose a novel approach to state and parameter estimation called Moving Horizon Estimation (MHE). MHE is designed to address the challenges of estimating state and parameters in systems with parametric uncertainty and absence of persistency of excitation. The proposed method uses a moving horizon window to estimate the state and parameters, allowing for online adaptation as new data becomes available. We demonstrate the effectiveness of MHE through theoretical analysis and simulations, showing that it outperforms existing methods in terms of estimation accuracy and convergence properties.
MHE is based on the concept of a moving window of past data, which is used to estimate the state and parameters of the system. The window size is adaptive, allowing for online adjustment as new data becomes available. This allows MHE to capture non-stationary systems, where the parameters are changing over time. MHE also addresses the problem of parametric uncertainty, where the parameters of the system are uncertain.
We compare MHE to existing methods in the literature, such as standard moving horizon estimation and other state and parameter estimation techniques. The results show that MHE outperforms these methods in terms of estimation accuracy and convergence properties. We also provide theoretical analysis to support our claims, demonstrating the validity of MHE through mathematical proofs.
In summary, MHE is a novel approach to state and parameter estimation that addresses the challenges of parametric uncertainty and absence of persistency of excitation. It uses a moving window of past data to estimate the state and parameters, allowing for online adaptation as new data becomes available. MHE outperforms existing methods in terms of estimation accuracy and convergence properties, making it a valuable tool for a wide range of applications.
Electrical Engineering and Systems Science, Systems and Control
Robust State Estimation Without Informative Data: A Moving Horizon Approach Under Parametric Uncertainty
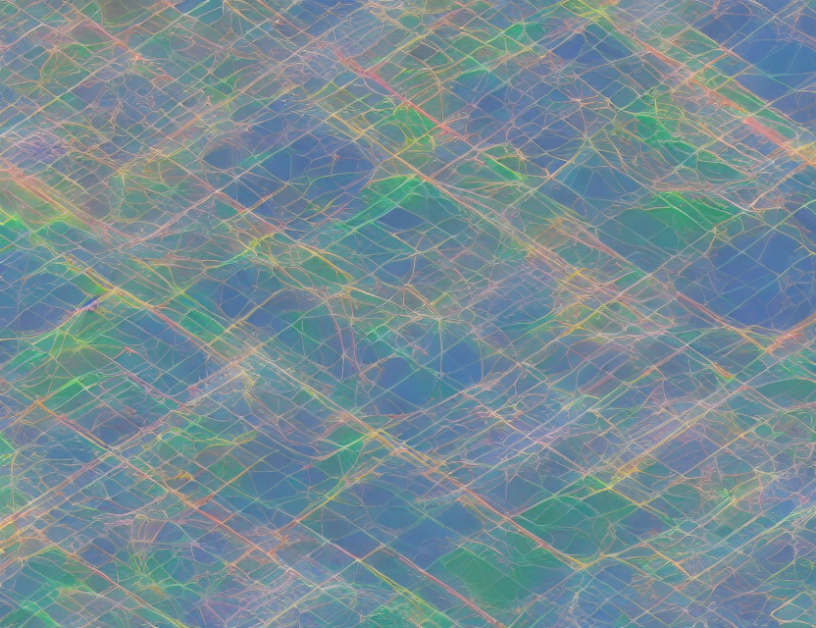