In this article, we explore the concept of data-driven predictive control, which is becoming increasingly popular in control design. Unlike traditional control methods that rely on mathematical models, data-driven approaches use real-world data to identify patterns and make predictions about future behavior. This new perspective offers several advantages, including improved accuracy and flexibility.
First, we define the terms model-based and model-free approaches in the context of data-driven predictive control. Model-based methods assume some knowledge of the underlying system, while model-free approaches rely solely on data. We show that in a linear, time-invariant setting, we can classify these approaches based on their relationship to the available data.
Next, we discuss the importance of considering the cost of the predictive control algorithm. In asymptotic statistical analysis, it is often necessary to assume equality up to order 1/√N, but in this context, we need to make sure that certain approximations have an effect that can be neglected compared to the dominating terms, which are of order O(1/N).
We then present a new perspective on data-driven predictive control, focusing on the idea of using a feasible future input sequence to minimize a user-defined cost function. We show how this approach can be applied in practice by solving an optimization problem at each time step and discarding the remaining optimal inputs.
Finally, we highlight the advantages of data-driven predictive control, including improved accuracy and flexibility. By using real-world data to inform our predictions, we can better handle complex systems and uncertainties. However, we also acknowledge that this approach is not without limitations, and further research is needed to fully realize its potential.
In summary, this article provides a comprehensive overview of data-driven predictive control, including its definition, advantages, and limitations. By using real-world data to inform our predictions, we can improve the accuracy and flexibility of control systems, opening up new possibilities for automation and optimization.
Electrical Engineering and Systems Science, Systems and Control
Data-Driven Predictive Control: A Model-Free Approach
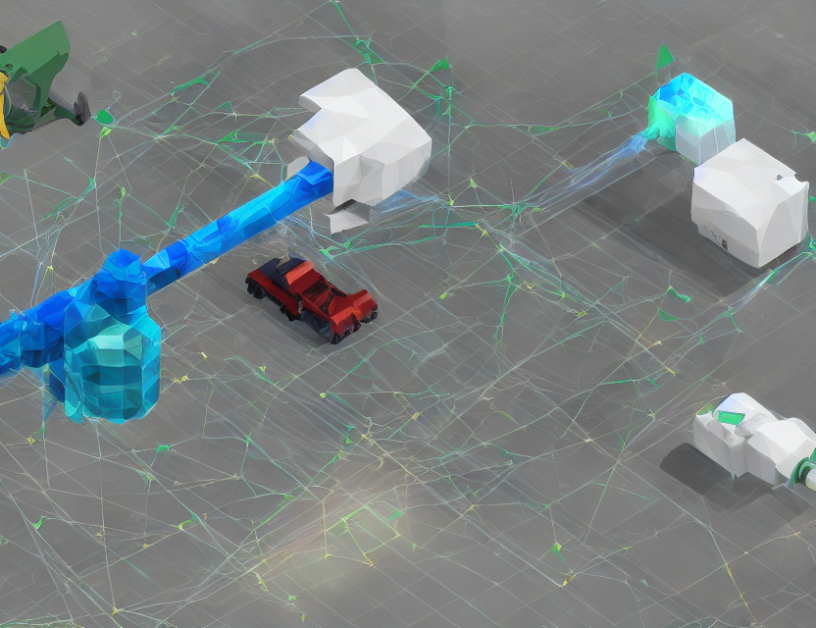