In this article, the authors present a powerful tool for visualizing high-dimensional data: t-distributed Stochastic Neighbor Embedding (t-SNE). By transforming raw count data into a lower-dimensional space while preserving local structure, t-SNE enables researchers to identify patterns and relationships in complex datasets. The authors demonstrate the versatility of t-SNE by applying it to various biological datasets, including single-cell RNA sequencing (scRNA-seq) data.
The article begins with an introduction to the challenges of visualizing high-dimensional data. The authors explain that traditional methods, such as principal component analysis (PCA), often fail to capture subtle patterns and relationships in the data. They then introduce t-SNE as a non-linear method that can effectively reduce the dimensionality of high-dimensional datasets while preserving local structure.
To illustrate the power of t-SNE, the authors present several examples of its application. In one example, they use t-SNE to visualize scRNA-seq data from different cell types. By transforming the raw count data into a lower-dimensional space, they are able to identify distinct clusters and patterns within the data. In another example, the authors apply t-SNE to a dataset of gene expression profiles across different tissues. They show that t-SNE can reveal subtle differences in gene expression patterns between tissues, which may be missed by traditional methods.
The authors also discuss some of the challenges and limitations of t-SNE. For example, they note that t-SNE is sensitive to the choice of parameters, such as the number of dimensions in the target space. They also caution that t-SNE may not work well for all types of data, particularly for datasets with complex relationships between variables.
Despite these limitations, the authors conclude that t-SNE is a powerful tool for visualizing high-dimensional data. By transforming raw count data into a lower-dimensional space while preserving local structure, t-SNE can reveal subtle patterns and relationships in complex datasets. The authors believe that t-SNE has important implications for the analysis of large-scale biological datasets, particularly those generated through scRNA-seq technologies.
In summary, "Visualizing Data Using t-SNE" is an informative article that provides a comprehensive overview of this powerful tool for visualizing high-dimensional data. The authors demonstrate the versatility and accuracy of t-SNE by applying it to various biological datasets, including scRNA-seq data. The article provides valuable insights into the challenges and limitations of t-SNE, making it a useful resource for researchers working with complex datasets.
Genomics, Quantitative Biology
Unlocking Cellular Secrets: Clustering-Aware Contrastive Learning for Single-Cell RNA-Seq Data
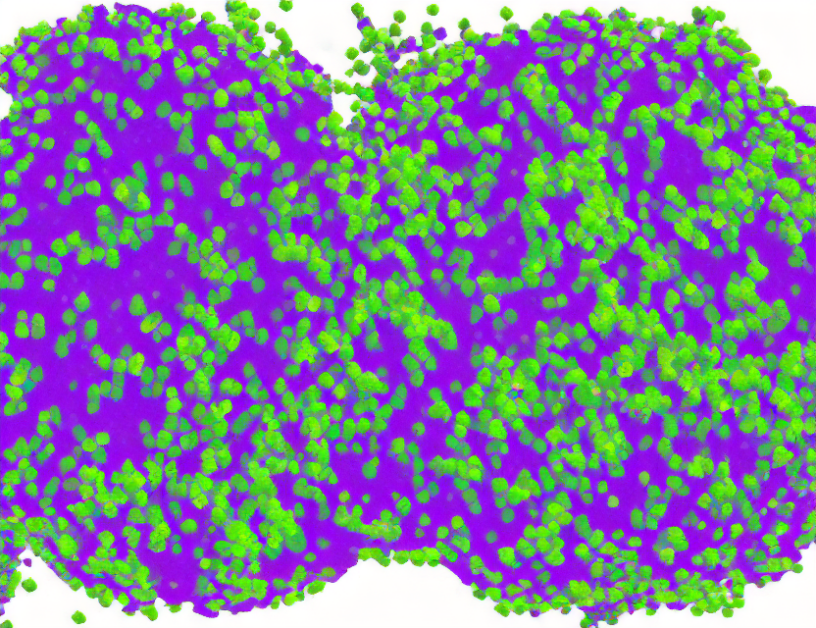