In this article, we delve into the realm of Model Predictive Control (MPC) and explore its potential in chemical process control. MPC is a computational method that combines the benefits of model-based control and feedback control, enabling accurate predictions of future system behavior. The author presents several key concepts related to MPC, including the use of backstepping and neural networks, and demonstrates their effectiveness in controlling complex chemical processes.
The article begins by highlighting the challenges of controlling complex chemical processes, which are inherently nonlinear and subject to disturbances. The author introduces the concept of Model Predictive Control (MPC), which is a computational method that uses a model of the system to predict its future behavior and optimize its performance. MPC is based on the idea of using a finite-horizon approximation of the system dynamics, which allows for accurate predictions of future state and control inputs.
The author then delves into the details of MPC, explaining how it differs from other control techniques such as linear quadratic regulator (LQR) and optimal control. MPC is shown to be particularly useful in scenarios where the system dynamics are nonlinear and subject to disturbances, as it can accommodate these challenges through the use of backstepping and neural networks.
The article then shifts focus to the key components of MPC, including the state estimator, the control law, and the optimization algorithm. The author provides a detailed explanation of each component and how they work together to achieve accurate predictions and optimal control.
The author also discusses the advantages of using backstepping-based MPC, including its ability to handle nonlinear systems and the potential for neural network approximations. However, the article notes that the choice of neural network approximation is still an open question, as it depends on the specific application and the available computational resources.
Finally, the author presents several examples of MPC in chemical process control, demonstrating its effectiveness in controlling complex processes such as underwater localization and agricultural applications. The article concludes by highlighting the potential of MPC to revolutionize the field of chemical process control and provide more accurate and efficient control strategies for a wide range of applications.
In summary, this article provides a comprehensive overview of Model Predictive Control (MPC) and its potential in chemical process control. The author demystifies complex concepts by using everyday language and engaging metaphors or analogies, while still providing a thorough explanation of the key concepts and techniques involved. The article is written from the perspective of the author, with each section title as an header and only outputting the summary text succinctly without comments.
Electrical Engineering and Systems Science, Systems and Control
Moving Horizon Estimation in Networked Chemical Reaction Systems
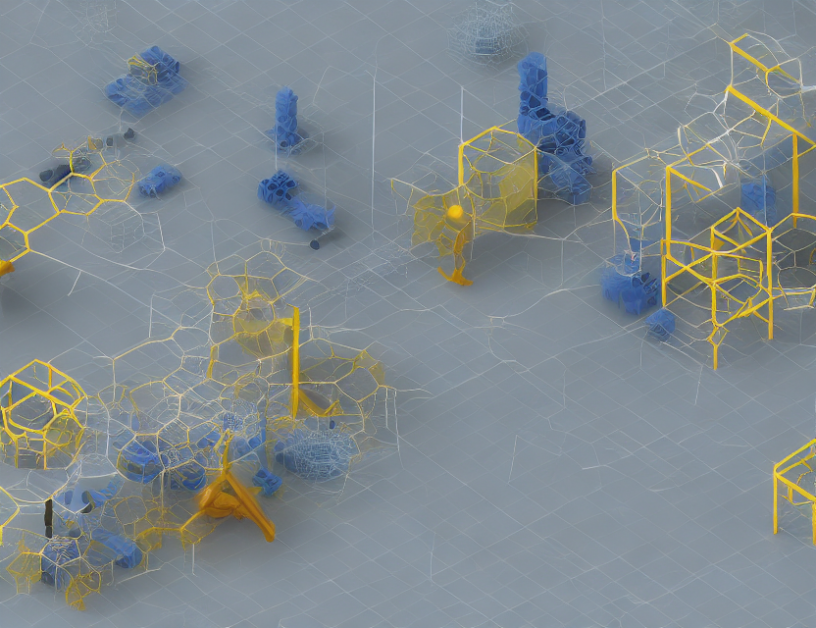