In the field of pathology, there is a growing interest in using artificial intelligence (AI) to analyze medical images. However, one of the major challenges in this area is the lack of transparency and interpretability of AI models, making it difficult to understand the reasoning behind their predictions. To address this issue, researchers have proposed the Vision Language Foundation (VLF) concept, which leverages collective knowledge from platforms such as medical Twitter to train AI models that can analyze medical images more effectively.
The VLF approach is based on a novel framework called Pathology Language-Image Pretraining (PLIP), which combines visual and textual data to create a unified representation of medical images. This allows the AI model to learn both the visual features of the image and the context provided by the accompanying text, leading to improved accuracy and interpretability.
To evaluate the performance of PLIP, researchers have conducted experiments on several benchmark datasets, including those containing histopathology slides. The results demonstrate that PLIP can achieve exceptional performance in zero-shot classification, outperforming traditional AI models that rely solely on visual features.
Furthermore, the VLF framework is not limited to image analysis but can also be applied to other medical applications, such as predicting patient outcomes based on medical images. The potential of this approach is substantial, as it could enable more accurate and efficient diagnosis, treatment, and monitoring of diseases.
In summary, PLIP represents a significant advancement in the field of pathology AI by providing a framework that can effectively manage the complex interplay between visual and textual data. By leveraging collective knowledge from platforms such as medical Twitter, PLIP can overcome the limitations of limited data availability and enable pioneering research in this domain. With its exceptional performance in zero-shot classification and potential applications beyond image analysis, PLIP holds great promise for improving healthcare outcomes.
Electrical Engineering and Systems Science, Image and Video Processing
Uncovering Adversarial Vulnerabilities in Medical AI Models
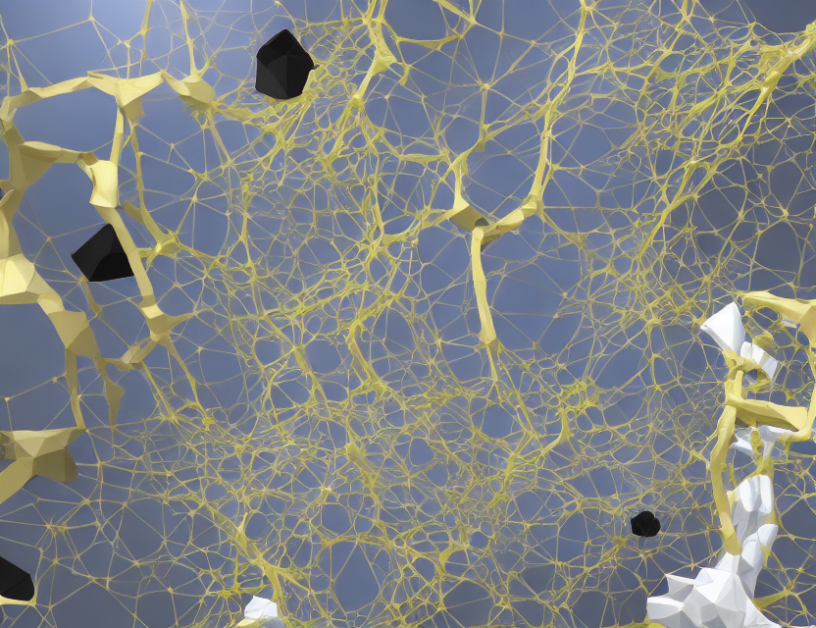