Human-like perception is a significant challenge in developing multimodal AI systems that can process and understand visual content as humans do. Researchers have developed various strategies to guide language models (LMMs) in extracting essential information from visual content, but they still fall short of emulating human perception. One major obstacle is the loss of image detail when using natural language, which is less precise than visual data. Additionally, understanding relationships between multiple image inputs can be challenging, requiring explanations of both individual elements and their spatial and contextual ties.
To address these issues, researchers have developed multimodal prompting strategies to help LMMs comprehend essential information from images. However, these methods still struggle with complex visual information, such as subtle lighting shifts or intricate patterns, which require extensive verbal descriptions. Moreover, matching images with text requires models to precisely extract information from images and match it with the appropriate text description.
The Winoground dataset is commonly used for testing multimodal AI systems’ ability to reason with both vision and language. The test includes three distinct metrics that evaluate different aspects of a model’s performance, including its ability to accurately select captions for images and identify the correct image when provided with a caption. The composite score integrates these two metrics, ensuring a comprehensive assessment of a model’s abilities.
In summary, emulating human-like perception in multimodal AI systems is a complex challenge that requires addressing both the loss of image detail in natural language and the difficulty in understanding relationships between multiple image inputs. While researchers have developed strategies to guide LMMs in extracting essential information from visual content, further advancements are needed to enable these models to emulate human-like perception.
Computer Science, Computer Vision and Pattern Recognition
Accurate Analysis of Image Captions with CoT-Based Methods
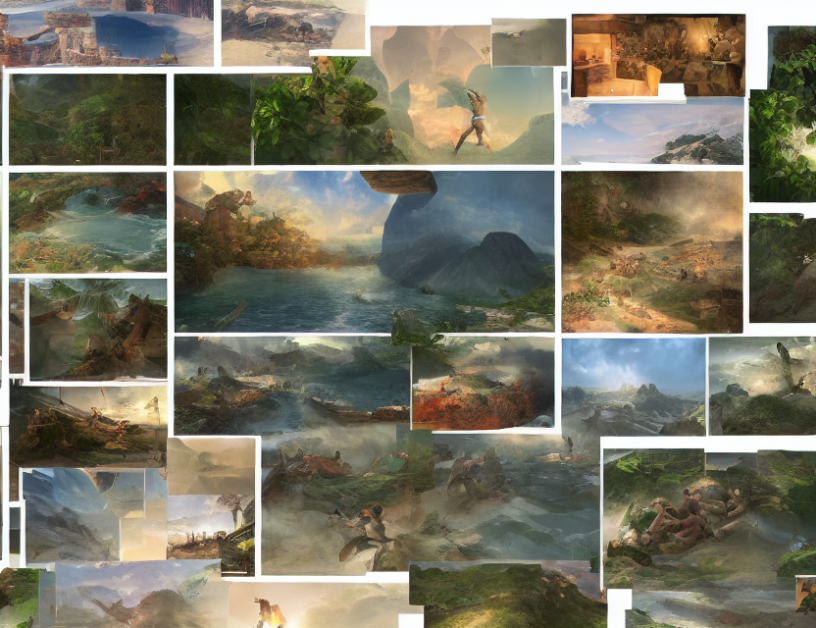