In this article, we present SimpleX, a simple yet powerful content-based filtering (CBF) model that outperforms state-of-the-art models by a large margin. Developed based on the mechanism of Matrix Factorization (MF), SimpleX incorporates additional inputs to better capture user behaviors and introduces an aggregation layer for behavior sequence aggregation. The key innovation of SimpleX lies in its ability to unify various aggregation methods, making it a versatile architecture that can be applied to any other aggregation method.
We evaluate the performance of SimpleX on three main datasets and compare it with existing models, including YouTubeNet, ACF, and PinSage. Our results show that SimpleX consistently outperforms these models, demonstrating its effectiveness in modeling user behaviors. We also perform parameter analysis and efficiency evaluation to further validate the simplicity and strength of SimpleX.
To create a comprehensive understanding of SimpleX, we analyze its relationships with existing CBF models and demonstrate its ability to unify various aggregation methods. By doing so, we aim to provide insights into why SimpleX performs well and how it can be applied in future research.
In summary, SimpleX is a simple yet powerful content-based filtering model that offers a strong baseline for future research. Its versatility and ability to capture user behaviors make it an ideal choice for applications where CBF is essential.
Computer Science, Information Retrieval
SimpleX: A Strong Baseline for Collaborative Filtering
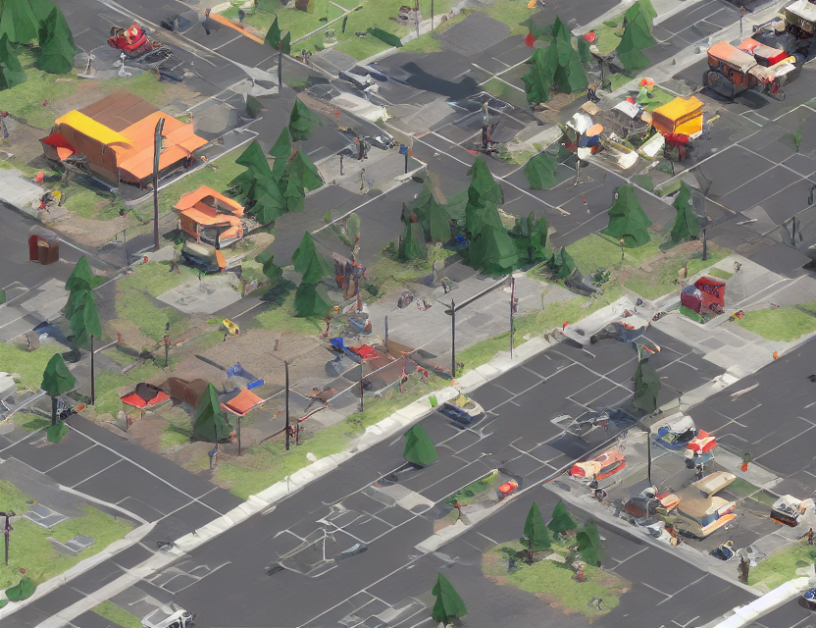