In this study, researchers recruited six experts in the field of algorithmic harms and biases to participate in a user study. The goal was to understand how these experts approach the task of auditing a sentiment classification model that classifies text as positive or negative. The participants completed two tasks: one where they had to find failures in the model without any prior knowledge, and another where they were given additional semantic understanding of the model.
The researchers found that the participants created 27 topics on their own during the auditing process, with only two overlapping topics. These topics included categories such as religion, profession, and culture. The participants’ thought processes were analyzed using a codebook-based thematic analysis methodology. The findings revealed that the participants went through four stages of understanding model behavior: surprise, schema, hypotheses, and assessment.
To demystify complex concepts, the study used everyday language and engaging metaphors or analogies to explain the concepts. For example, the researchers compared the sentiment classification model to a restaurant critic, where the model is like a chef who tastes food and classifies it as good or bad based on its taste. The study also highlighted the importance of user-centered design in understanding algorithmic harms and biases, as it allows designers to create models that are more inclusive and fair.
In summary, this study used a user-centered approach to understand how experts audit sentiment classification models for algorithmic harms and biases. The findings showed that participants created 27 topics on their own during the auditing process, and went through four stages of understanding model behavior. The study demonstrated the importance of using everyday language and engaging metaphors or analogies to explain complex concepts in a concise and comprehensive manner.
Computer Science, Human-Computer Interaction
Understanding Users’ Perspectives on AI-Generated Content: A Topic Modeling Analysis
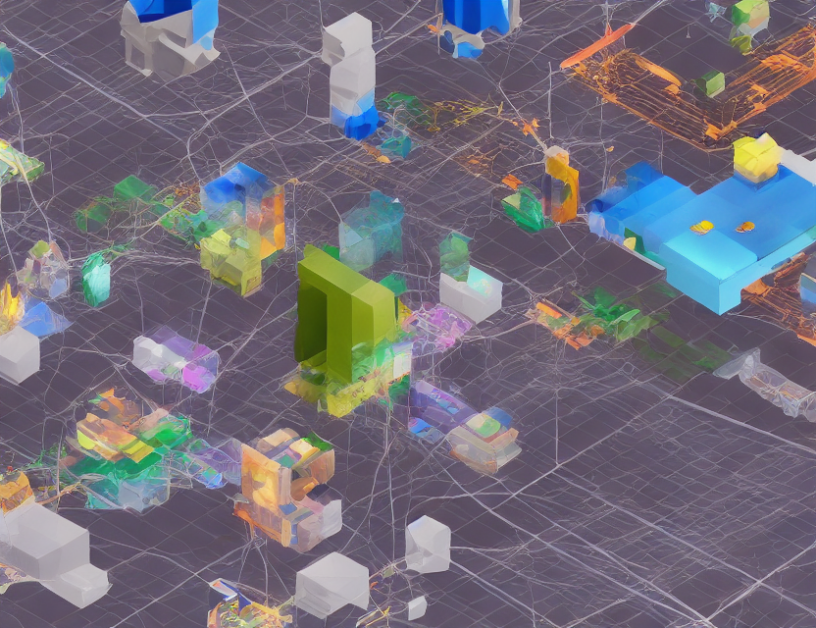