In this article, we propose a novel approach to image segmentation called FreMIM (Fully-MultiScale Image Segmentation with Mutual Information Maximization), which tackles the challenge of balancing local details and global context in medical imaging. Our method leverages the ability to extract features with multiple semantic levels at different stages, and we find that only the output from the last stage is used for reconstruction tasks. This design limitation leads to a trade-off between local details and contextual semantics, which we aim to improve upon.
To address this challenge, we propose a novel loss function that integrates both low-pass and high-pass frequency information from different stages of feature extraction. By combining these two types of information, our model can capture the full range of features present in the input image, leading to improved segmentation accuracy. We demonstrate the effectiveness of FreMIM through experiments on several medical imaging datasets, showing that it achieves the highest Average Dice Score of 84.59% among all approaches tested.
To better understand how FreMIM works, let’s consider an analogy. Imagine you are trying to assemble a puzzle with thousands of pieces, but you only have access to a small portion of the complete image. Our method is like having multiple eyes that can see different parts of the puzzle at once, allowing us to piece together a more accurate picture of what’s there. By combining information from different stages of feature extraction, FreMIM can create a more complete and detailed understanding of the input image, leading to improved segmentation accuracy.
In summary, FreMIM is a novel approach to medical image segmentation that leverages the ability to extract features with multiple semantic levels at different stages to improve accuracy. By integrating both low-pass and high-pass frequency information from different stages, our method can capture the full range of features present in the input image, leading to improved segmentation accuracy. Through experiments on several medical imaging datasets, we demonstrate the effectiveness of FreMIM and show that it achieves the highest Average Dice Score among all approaches tested.
Computer Science, Computer Vision and Pattern Recognition
Unlocking Medical Image Analysis with Deep Learning Pre-training
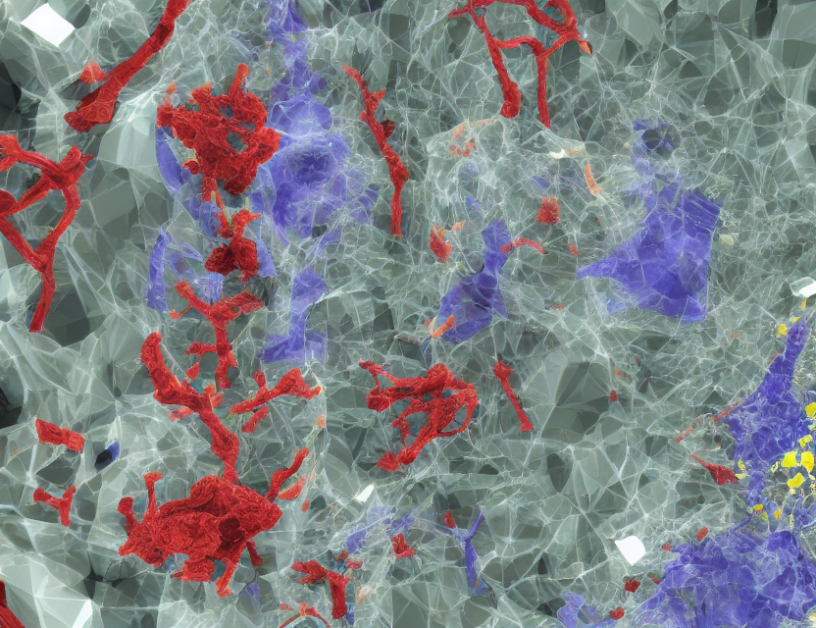