As a developer, you may have heard about "sustainable machine learning" (ML) but struggle to understand what it entails. This article provides a comprehensive explanation of the concept and its significance in the field of ML development.
Firstly, sustainable ML refers to the practice of developing ML models that are energy-efficient, environmentally friendly, and socially responsible. The author highlights the importance of reporting carbon emissions associated with ML model development and usage to promote transparency and accountability. This is essential for organizations to understand their environmental impact and make necessary changes to reduce their carbon footprint.
The article also discusses related work in the area, including the need for standardized reporting guidelines and best practices for sustainable ML development. The author emphasizes that establishing such guidelines can improve the consistency and quality of energy data and context reported, leading to more accurate comparisons and conclusions.
To address the challenge of lacking standardized reporting practices for carbon emissions, the author suggests future research should focus on developing more systematic approaches to collecting and reporting data on carbon emissions associated with ML models. This will help improve conclusion validity and enable organizations to make informed decisions about their environmental impact.
In summary, sustainable ML is an essential aspect of responsible ML development that involves energy-efficient model creation, transparency in energy consumption and emissions, and socially responsible practices. By establishing standardized reporting guidelines and promoting more systematic data collection practices, organizations can ensure their ML models are environmentally friendly and ethical, contributing to a more sustainable future for all.
Computer Science, Machine Learning
Standardizing Energy Reporting in AI Development: A Key to Sustainable ML
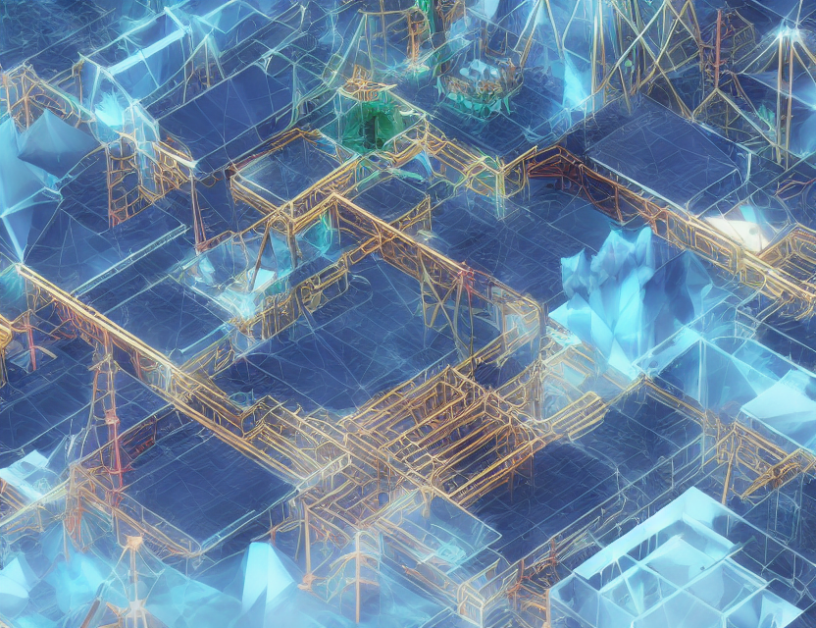