In this article, we explore the challenges of using machine learning (ML) in dynamic social systems where there are power imbalances between stakeholders. The main issue is that the metrics used to optimize ML models do not always align with social welfare. We examine this problem through various experiments on clinical datasets and show that the regret incurred by the widely used average treatment effect (ATE) estimator can be high, especially when there is a lack of information symmetry between stakeholders. To address this challenge, we propose using incentive-aware evaluation mechanisms to maximize welfare while accounting for the information asymmetry.
To understand the issue at hand, imagine you are trying to optimize a recipe for your favorite dish. You want to make sure it turns out perfect, but you also know that some ingredients can be quite expensive. In this scenario, you might decide to prioritize the cheaper ingredients over the more expensive ones to save money and still achieve a delicious meal. However, if you don’t consider the impact of your choices on the overall dish, you might end up with an inedible mess.
In ML, the objective is similar. We want to optimize our models to achieve the best possible outcomes, but we need to be mindful of the information asymmetry between stakeholders. If we don’t consider this issue, we risk optimizing metrics that don’t align with social welfare, leading to suboptimal outcomes.
To address this challenge, we propose using incentive-aware evaluation mechanisms. These mechanisms take into account the information asymmetry between stakeholders and ensure that the optimization process prioritizes the most important metrics for social welfare. It’s like having a personal chef who understands your preferences and budget constraints, optimizing the recipe to create the perfect dish while keeping costs under control.
We evaluate our approach through various experiments on clinical datasets and show that it can significantly reduce regret incurred by the ATE estimator. It’s like reducing the risk of overcooking or underseasoning a dish – with careful attention to detail, we can create an optimal outcome that satisfies everyone involved.
In summary, this article highlights the challenges of using ML in dynamic social systems with information asymmetry and proposes incentive-aware evaluation mechanisms to address these issues. By prioritizing the most important metrics for social welfare, these mechanisms can help optimize ML models while ensuring that they align with the goals of all stakeholders involved.
Computer Science, Machine Learning
Operationalizing Counterfactual Metrics: Incentives, Ranking, and Information Asymmetry
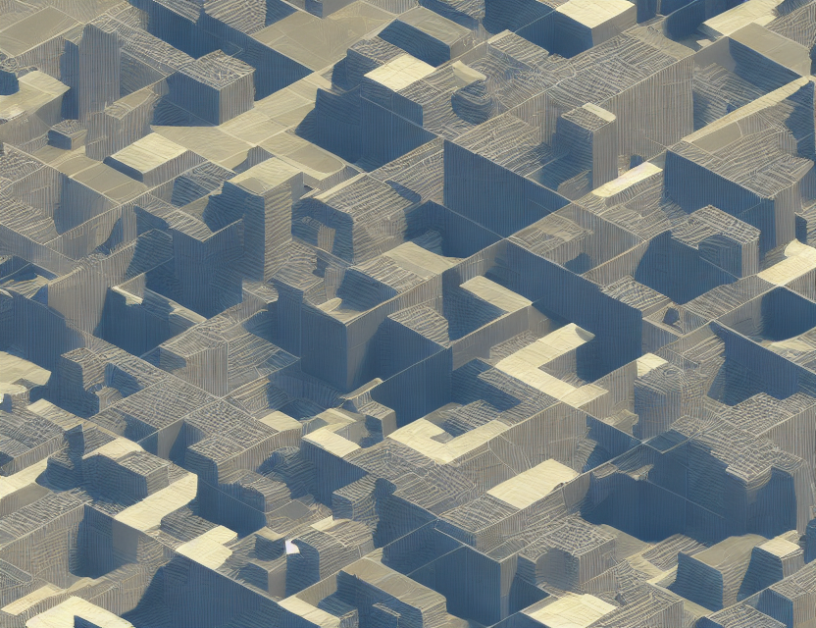