Compositionality is a crucial aspect of language, allowing us to communicate complex ideas by combining simpler components. In the context of Stable Diffusion models, compositionality refers to the ability of these models to generate images that are more than just a collection of individual objects. However, this task is not without its challenges, as the models can struggle to represent certain concepts, such as object viewpoints and parts, and often produce failures in their outputs.
To address these challenges, the authors propose several approaches, including incorporating symmetry detection in the evaluation step and using two different strategies to improve the model’s understanding of complex actions and concepts. They also provide a detailed analysis of individual failure cases, revealing that many of these failures are due to adjectives, specific motions, or salient features in words, which are equally important but often overlooked.
The authors use several metrics to evaluate the performance of their proposed approaches, including the failure generation rate (FGR(H)) per category. They find that DeepFloyd, a model that incorporates more fine-grained understanding of numbers and fractions, shows better performance in these areas compared to Stable Diffusion V2.1. However, they also find that all models struggle to express object viewpoints and parts, and that the failure reasons tend to be different for each category.
In conclusion, the article provides a comprehensive analysis of compositionality in Stable Diffusion models, identifying the challenges and proposing several approaches to improve their performance. By using everyday language and engaging metaphors or analogies, the authors aim to demystify complex concepts and make the article accessible to an average adult reader.
Computer Science, Computer Vision and Pattern Recognition
Uncovering Hidden Failures in Text-to-Image Synthesis: A Closer Look at CLIP
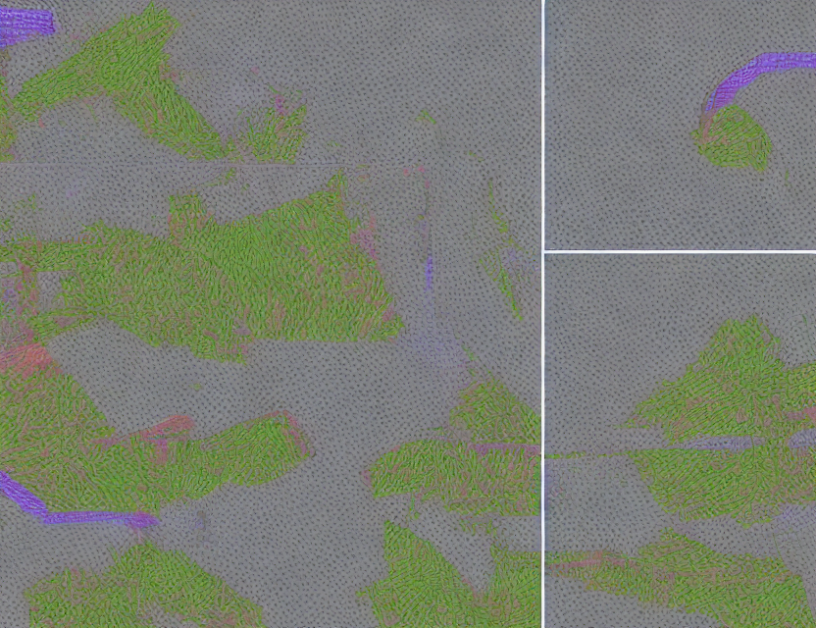