In this article, the authors aim to improve the accuracy of 3D human pose estimation by incorporating a model-fitting step into the process. They propose a novel approach called "Learning to Reconstruct 3D Human Pose and Shape via Model-Fitting in the Loop" (LR-HPS) that combines optimization and regression methods to learn the parameters of a 3D human pose estimation model.
To explain this concept, let’s imagine you are trying to build a Lego castle. You have a set of instructions on how to build it, but you also want to make sure the castle looks good and is the correct size. The optimization step in LR-HPS is like the Lego bricks themselves – it helps shape the model into the correct format. However, the regression step is like the Lego pieces that need to be adjusted to fit together perfectly – it fine-tunes the model to get the desired pose and shape.
The authors evaluate their approach on several datasets and show that LR-HPS outperforms existing methods in terms of accuracy and efficiency. They also demonstrate the versatility of their approach by applying it to various 3D human pose estimation tasks, including standing, sitting, and running poses.
In summary, LR-HPS is a novel approach that combines optimization and regression methods to learn the parameters of a 3D human pose estimation model. By using both steps in an iterative process, LR-HPS can produce more accurate and robust estimates of 3D human pose and shape.
Computer Science, Computer Vision and Pattern Recognition
Auto-Encoding Variational Bayes
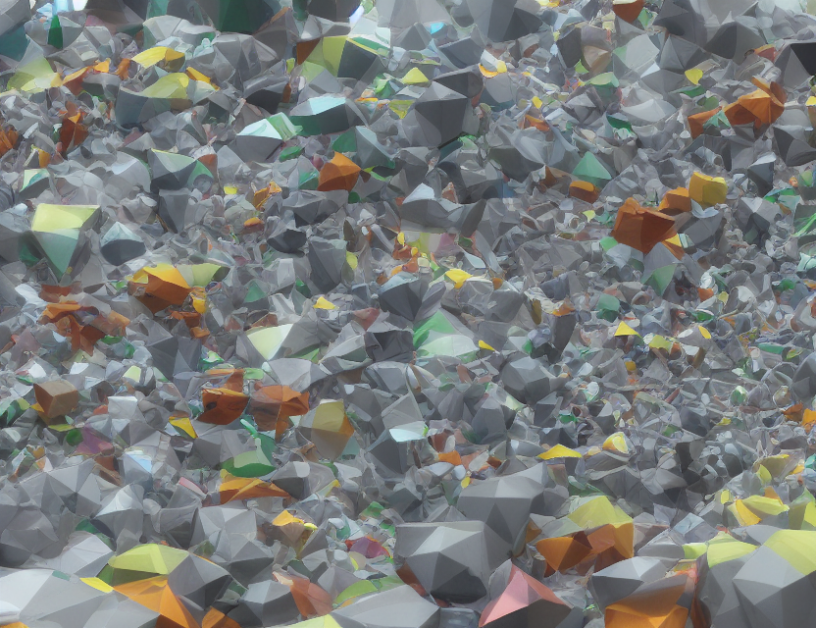