In this article, we delve into the realm of hypergraphs, a structure that extends the classic graph by allowing multiple relationships between nodes. We explore how this additional flexibility enables the modeling of complex social phenomena, such as opinion formation, which can be better understood by analyzing the interplay between individuals and their communities.
The authors highlight the limitations of traditional graph models in capturing the intricate nature of these interactions and propose a novel approach based on hypergraph p-Laplacians and scale spaces. By leveraging these tools, they demonstrate how to analyze information flow in social networks, focusing on the role of opinion leaders who have a profound impact on shaping public opinion.
To illustrate their points, the authors provide examples from real-world scenarios, such as analyzing the spread of misinformation on social media platforms. They show how hypergraph models can help uncover the underlying structure of these networks and identify key individuals who play a crucial role in molding public opinion.
Throughout the article, the authors strive to make complex concepts accessible by using everyday language and engaging analogies. For instance, they describe the hypergraph structure as a "web" of relationships, where each node represents an individual and the arcs represent the connections between them. They also compare the p-Laplacian matrix to a "filter" that removes noise from the signal, allowing for more accurate analysis of information flow.
In summary, this article provides a comprehensive overview of hypergraphs and their applications in modeling social phenomena, particularly opinion formation. By leveraging novel mathematical tools such as hypergraph p-Laplacians and scale spaces, researchers can gain a deeper understanding of how complex networks operate and identify key individuals who shape public opinion. The authors’ use of simple language and engaging analogies makes the concepts more accessible to a wider audience, making this article an excellent resource for anyone interested in social network analysis.
Computer Science, Social and Information Networks
Numerical Study of Opinion Formation in Hypergraphs
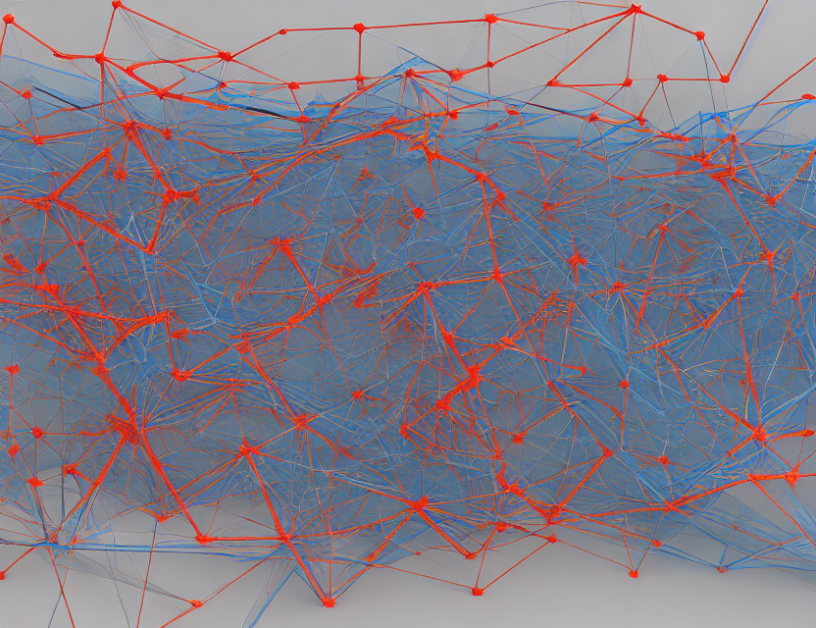