Imagine you’re browsing a website for movie recommendations. You see a list of movies, but you want more personalized suggestions that match your interests. Enter pre-trained models, which are like superheroes in the world of recommendation systems. These models have already learned to understand text from various sources, such as movie descriptions and user reviews.
The authors propose using these pre-trained models to improve the accuracy of personalized recommendations. They call this approach "Pre-Rec," which stands for "pre-training for recommendation." By combining Pre-Rec with Bayesian inference, they can better capture the complex relationships between users, items, and domains.
The authors explain that traditional methods for personalized recommendations rely on a single model to learn from all users and items. However, this approach can lead to inaccurate recommendations when the model is not exposed to enough data or when it encounters new users or items. Pre-Rec addresses these issues by using pre-trained models to capture domain-specific knowledge and improve the generalization of the recommendation system.
The authors demonstrate the effectiveness of Pre-Rec through experiments on a real-world dataset. They show that their approach outperforms traditional methods in terms of accuracy and efficiency. They also provide insights into how different factors, such as user interests and item popularity, affect the recommendations.
In conclusion, pre-trained models are like superheroes in personalized recommendation systems. By combining them with Bayesian inference, the authors have developed a more accurate and efficient approach to recommend items that match users’ interests. This innovative approach has significant implications for applications such as online shopping, social media, and entertainment platforms.
Computer Science, Information Retrieval
Causal Debiasing in Pre-trained Recommender Systems
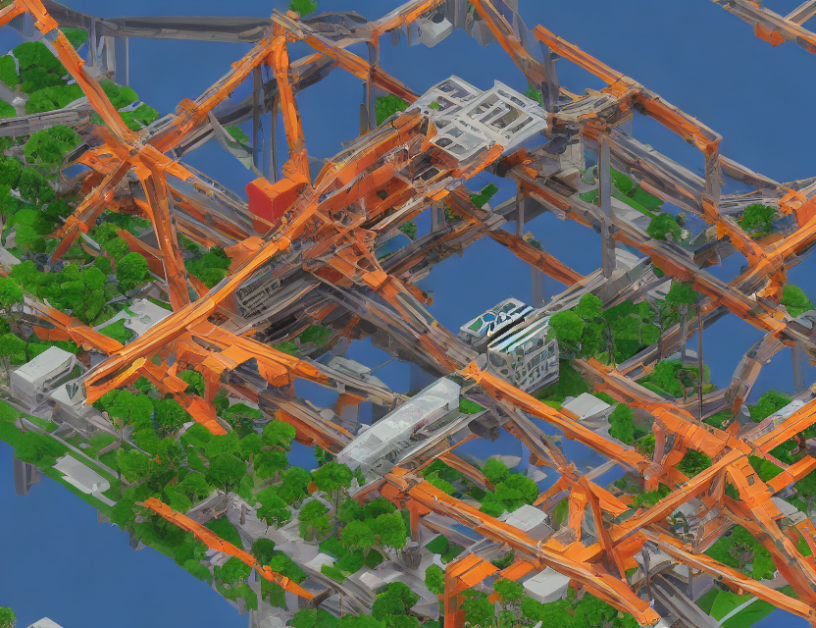