In this article, we present a novel approach to generating high-quality 3D shapes using natural language descriptions as input. Our method builds upon recent advances in transformer-based architectures and introduces a new cross-attention module that enables the model to capture localized information from the text. This allows for the generation of diverse and structured 3D shapes, surpassing previous works in terms of both shape and color quality.
To evaluate the effectiveness of our approach, we conduct ablation studies and comparison experiments with state-of-the-art methods. Our results show that our model consistently outperforms existing works across various metrics, demonstrating its superior generative quality and text consistency.
Our approach is different from traditional 3D shape generation methods in that it does not rely on predefined templates or meshes. Instead, the model learns to generate shapes directly from natural language descriptions, which can be as simple or complex as desired. This democratizes the creation of 3D shapes and makes it accessible to a wider range of users.
To generate 3D shapes, our model uses a combination of transformer encoders and decoders. The encoder processes the input text description and generates a set of contextualized features, while the decoder uses these features to construct the 3D shape. The cross-attention module in our decoder allows the model to focus on specific parts of the text when generating each 3D feature, ensuring that the generated shape is consistent with the input description.
One of the key innovations of our approach is the use of word-level cross-attention modules. These modules allow the model to embed localized information from the text into the feature space, enabling the generation of shapes with fine-grained details. This is particularly useful for generating complex shapes with multiple components or structures.
In summary, our paper presents a significant breakthrough in the field of 3D shape generation by developing a novel approach that uses natural language descriptions as input. Our method has the potential to revolutionize various fields such as computer-aided design, virtual reality, and artistic creation. With its ability to generate diverse and high-quality 3D shapes directly from text, our approach could open up new possibilities for creativity and innovation in these areas.
Computer Science, Computer Vision and Pattern Recognition
Enhancing Generative Quality of Shape and Color through Text-Guided Modification
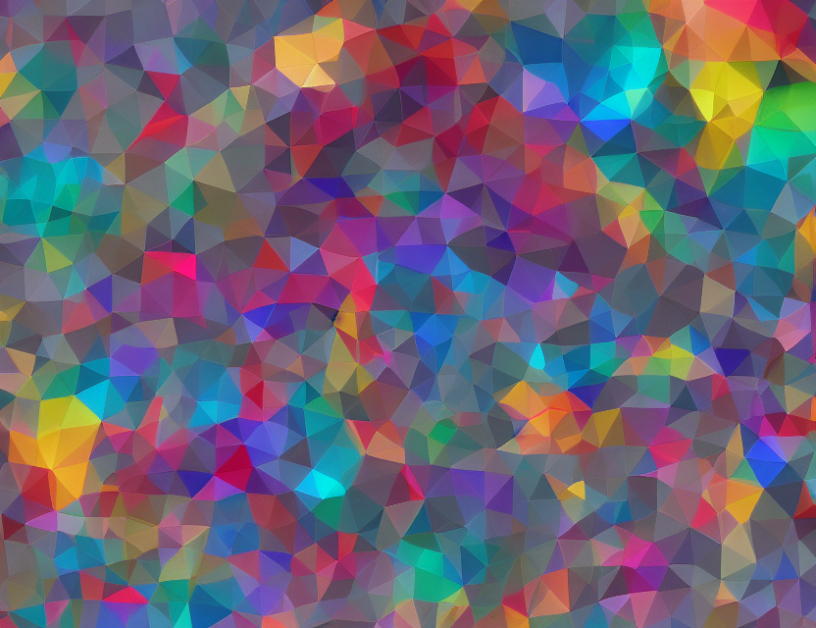