In this article, researchers present a comprehensive benchmark dataset called "AnyWord-3M" for evaluating the quality of text-to-image synthesis models. The dataset consists of 3 million images, each with a corresponding text description, and covers various topics such as objects, scenes, and styles. To ensure diverse and high-quality images, the authors use a novel sampling strategy that selects up to 5 text lines from each image and ensures a line count of at least 20 characters in each image.
The dataset is divided into four subsets: Wukong, LAION, OCR datasets, and total. Wukong contains 3 million images with captions, while LAION has 1.54 million images without captions. OCR datasets are used for training text recognition models, while the remaining images form the total subset.
The authors analyze the statistics of the dataset and reveal that the line count distribution follows a Poisson distribution. They also show that the majority of images have fewer than 20 characters in their text descriptions, indicating that simpler captions are more common. Furthermore, they demonstrate that their sampling strategy can cover most cases in the dataset.
To evaluate the quality of the images generated by text-to-image synthesis models, the authors use a series of metrics such as line count, mean lines per image, and unique characters per image. They show that their benchmark is large enough to train robust models with high accuracy.
In conclusion, AnyWord-3M is an extensive dataset that provides a valuable resource for researchers developing text-to-image synthesis models. The diverse range of images and captions ensures the quality of the generated images, while the sampling strategy guarantees a consistent distribution of images across various topics. By using this dataset, researchers can evaluate and improve their models’ performance on generating high-quality images from text descriptions.
Computer Science, Computer Vision and Pattern Recognition
Text-to-Image Synthesis: A Diffusion-Based Approach for Multilingual Text Generation and Editing
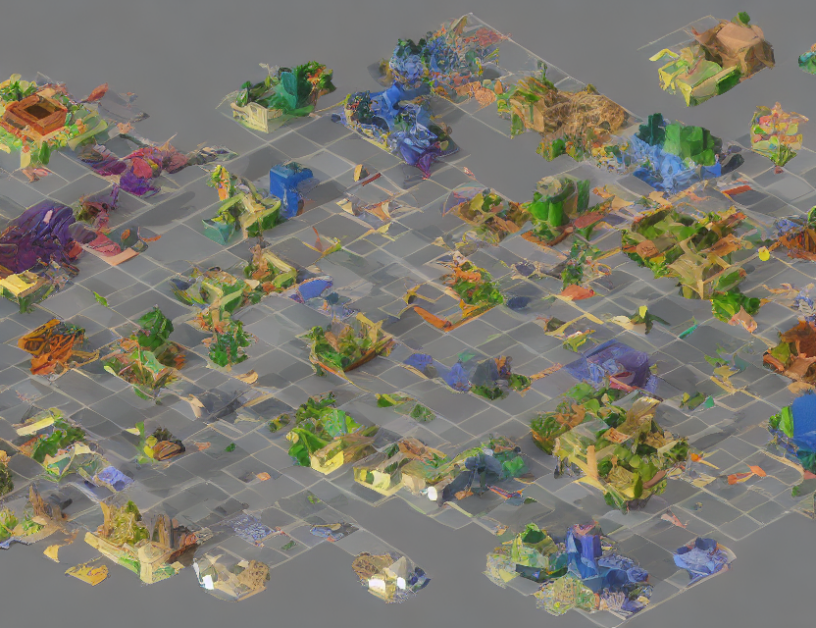