In the field of Artificial Intelligence (AI), fairness is a crucial aspect that ensures machines do not discriminate against certain groups of people. However, the concept of fairness can be complex and difficult to understand, leading to a "formalism trap" where simple statistical measures are used without considering the broader context. This article aims to demystify fairness in AI by exploring its various dimensions and highlighting the need for interdisciplinary collaboration among experts in different fields.
The authors argue that fairness is not just about complying with ethical values but rather a complex concept that involves multiple dimensions, including procedural, contextual, and contestable aspects. They emphasize that statistical measures alone cannot capture these subtle dimensions and must be used in conjunction with other tools to provide a comprehensive understanding of fairness.
One of the key points highlighted in the article is the importance of considering sensitive information, such as political or religious opinions, when evaluating fairness in AI systems. The authors note that simply being "unaware" of such information is not enough to prevent discrimination and emphasize the need for a more nuanced understanding of fairness.
The article also discusses the concept of "fair-washing," where techniques are used to promote false perceptions of compliance with ethical values, but this concept can be extended to fair-AI techniques as well.
To address these challenges, the authors advocate for interdisciplinary collaboration among experts in different fields, including statisticians, AI experts, ethicists, and legal experts. They suggest that this collaborative approach could lead to a shared vocabulary and set of working notions, potentially resolving the ambiguities surrounding fairness in AI.
In conclusion, the article demystifies fairness in AI by highlighting its complex dimensions and the need for interdisciplinary collaboration to address these challenges. By understanding the subtle aspects of fairness and how it interacts with sensitive information, researchers and practitioners can work towards developing more transparent and ethical AI systems that promote social good.
Artificial Intelligence, Computer Science
Unfair Algorithms: The Hidden Risks of Automated Decision-Making
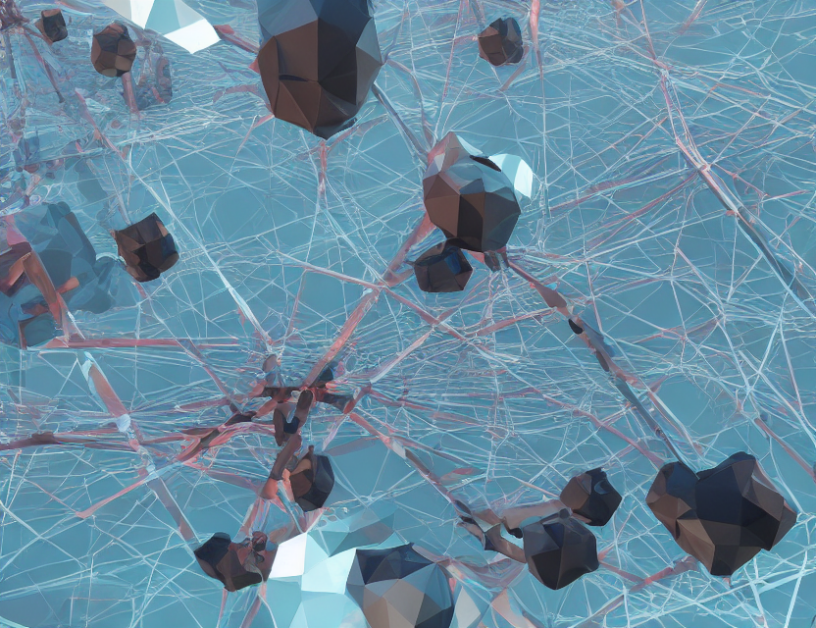