In this article, we delve into the fascinating world of visual grounding, which involves connecting images and captions in a way that helps machines understand their relationship. We explore how researchers have developed innovative techniques to improve the accuracy of image-text matching, including the use of contrastive learning and large-scale datasets.
The article begins by providing context on the existing methods for visual grounding, which typically involve training models to predict captions for images. However, these approaches often struggle with noisy or low-quality data, leading to suboptimal performance. To address this challenge, researchers have proposed a new paradigm called CLIP (Contrastive Language-Image Pre-training), which involves learning a shared representation space between images and captions using a dual-encoder approach.
One of the key innovations of CLIP is the use of kNN augmentation, which helps to reduce the impact of noisy data on the model’s performance. Additionally, the authors propose an additional self-supervision loss term to improve the robustness of the model against variations in image quality. The result is a significant improvement in zero-shot accuracy across various benchmarks, including Imagenet-1K, MS-COCO retrieval, and robustness against different image versions.
The article also discusses recent advancements in visual grounding, such as Uniclip and Bclip, which aim to unify the framework for contrastive language-image pre-training. These models have demonstrated excellent performance on various tasks, including image captioning, question answering, and visual generation.
To further improve the accuracy of image-text matching, researchers have explored the use of additional loss terms, such as consistency regularization and self-supervision. One promising approach is to impose additional regularization on the model’s weights to encourage it to produce more consistent predictions. Another strategy is to add a self-supervised loss term that encourages the model to generate accurate captions even when the image quality is poor.
Overall, the article provides a comprehensive overview of the latest developments in visual grounding and contrastive learning. By understanding these techniques and their applications, researchers can improve the accuracy of image-text matching and unlock new possibilities for computer vision and natural language processing.
Computer Science, Machine Learning
Mitigating Backdoors in ImageNet Classifiers Through Pre-training Objectives
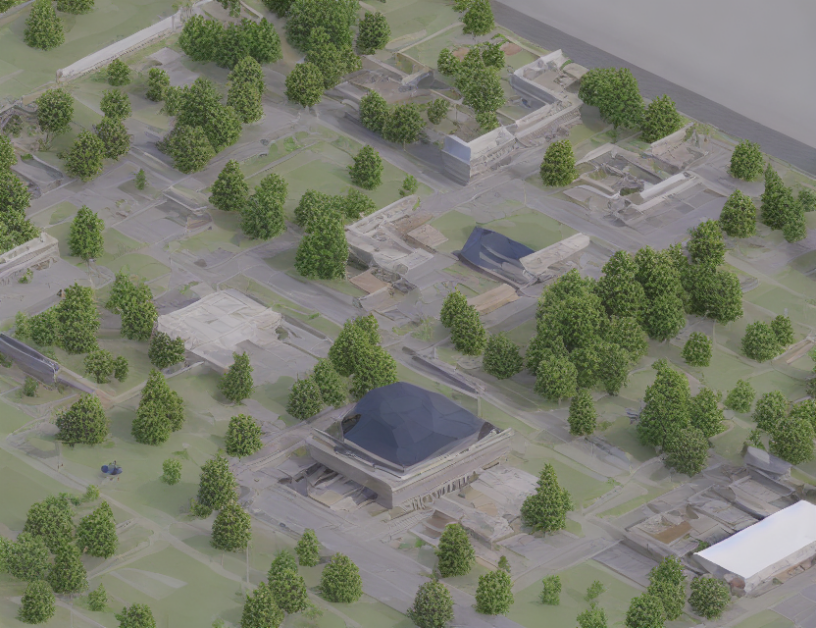