In this article, we explore a novel approach to image restoration called Cognitive Super-Resolution (CoSeR). Our method harnesses the power of cognition to enhance the process of enlarging low-resolution (LR) images. By generating cognitive embeddings that capture the underlying meaning and appearance of an LR image, we can leverage pre-trained text-to-image generation models to produce higher-quality super-resolved (SR) images.
To begin with, let’s understand why traditional SR methods struggle to handle real-world scenarios. The issue lies in the disparity between the image embedding and language embedding. While image features capture spatial details, language features encapsulate comprehensive information. As a result, a single language token may correspond to multiple subjects dispersed throughout an image. This makes it challenging for SR models to accurately restore image details without additional cues.
This is where cognition comes into play. Our CoSeR method starts by generating cognitive embeddings that capture the overall understanding of an LR image, including both scene semantics and appearance. These embeddings are then utilized to improve the SR process. By aligning the cognitive embedding with pre-trained text-to-image generation models, we can exploit their implicit prior knowledge, leading to enhanced image restoration capabilities.
Now, you might wonder how our approach differs from traditional SR methods. The key distinction lies in the use of cognition to infuse the SR process with a "top-down" cognitive process, mimicking human perception. Unlike previous work that relies solely on image priors or pre-trained models, CoSeR combines both bottom-up and top-down approaches for more accurate restoration.
In summary, our Cognitive Super-Resolution method offers a groundbreaking approach to enhancing image restoration capabilities. By leveraging cognition, we can better understand the underlying meaning and appearance of LR images, leading to higher-quality SR output. With CoSeR, we take a significant step towards bridging the gap between low-level image processing and high-level abstract cognition, opening up new possibilities for image restoration in real-world scenarios.
Computer Science, Computer Vision and Pattern Recognition
Efficient Image Captioning via Cognitive Adaptation
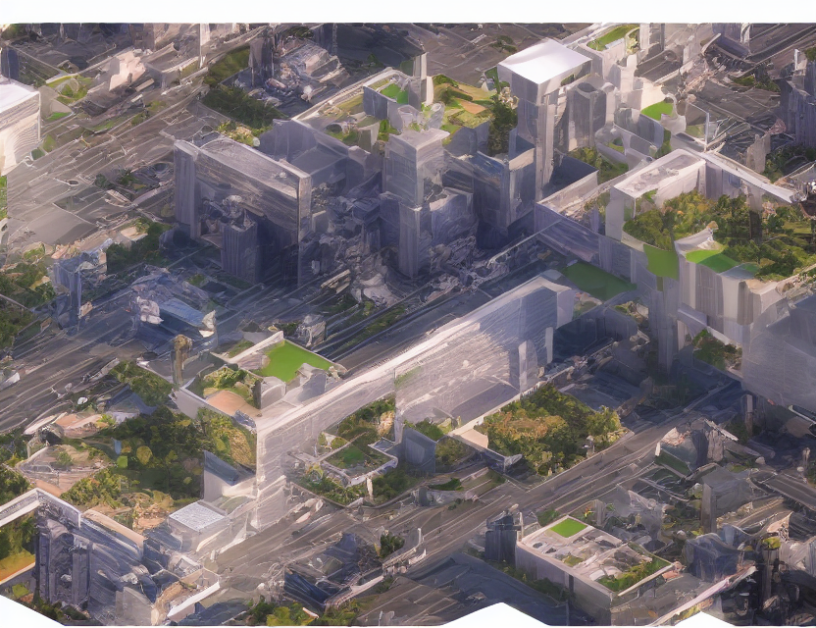