Carbohydrates are crucial biomolecules that play vital roles in various bodily functions. However, their structures and properties are complex, making it challenging to understand their functions. Traditional methods for determining carbohydrate structures rely on nuclear magnetic resonance (NMR) spectroscopy, which requires expertise and is limited by theoretical understanding.
To address these limitations, the article proposes using machine learning (ML) to analyze NMR data and predict carbohydrate structures. ML algorithms can process vast amounts of data quickly and accurately, demystifying complex concepts through everyday language and engaging metaphors. By integrating ML with NMR, researchers can gain a deeper understanding of carbohydrate structure-function relationships, which are essential for developing new drugs and therapies.
The article highlights the potential of two ML models, ComENet and DimeNet++, to predict carbohydrate structures from NMR data with high accuracy. These models can identify subtle structural features that are critical for understanding carbohydrate function. The authors also emphasize the importance of using extra features, such as chemical shifts and scalar coupling constants, to improve the accuracy of structure predictions.
The article further explores the limitations of current ML approaches in theoretical understanding and the need for better integration between ML and NMR. The authors acknowledge that ML models still require expert interpretation and domain knowledge, but they offer a promising opportunity to demystify carbohydrate structure-function relationships.
In summary, the article provides a comprehensive overview of the potential of ML in unlocking the secrets of carbohydrate structure and function. By combining NMR data with ML algorithms, researchers can gain a deeper understanding of these complex biomolecules, leading to new drug discoveries and therapies. While there are challenges to overcome, the article offers a hopeful outlook on the future of carbohydrate research and its impact on various fields of medicine.
Computer Science, Machine Learning
Advanced Materials for Biological and Environmental Applications
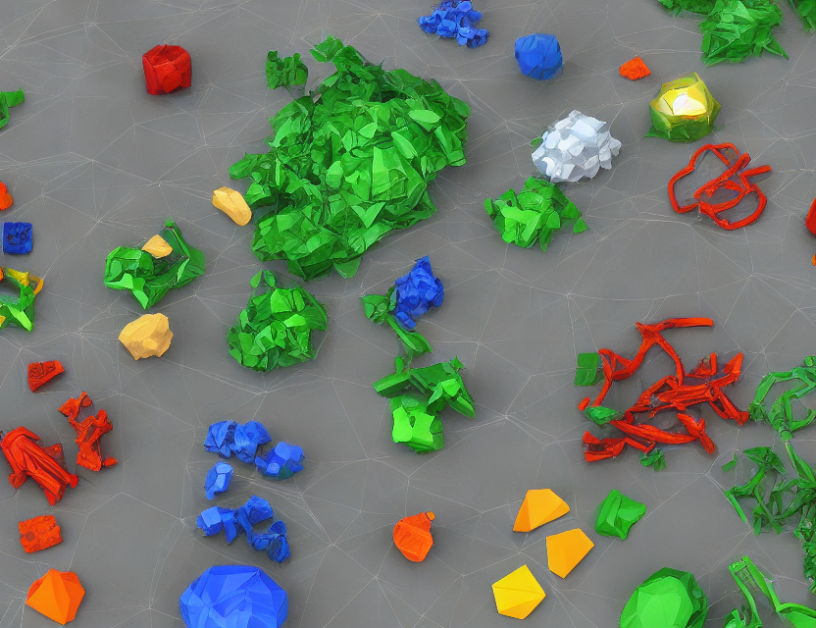