The article discusses the capabilities of two machine learning-based systems, High-MAST and Low-MAST, in identifying underlying sources and methodologies upon which results are based. High-MAST provides detailed information about the data used to gather clusters, including the clustering model, training data, and quality of the data. It also uses three main visualizations to enhance users’ understanding of the clusters. On the other hand, Low-MAST offers more details about a given cluster by clicking on it, but lacks information on how the titles or summaries are calculated. Both systems have limitations in assessing the accuracy of the topics and summaries since underlying sourcing information and raw data are not included.
The article also discusses trust in AI-DSS and validation of MAST, which is crucial for ensuring the accuracy and reliability of the results. High-MAST includes a datasheet with information on the clustering model, training data, and quality of the data used to derive the clusters. However, Low-MAST does not provide a similar level of detail or transparency in its visualizations.
In terms of incorporating visual information, both systems use visualizations to enhance users’ understanding of the clusters. High-MAST uses three main visualizations, while Low-MAST uses two. These visualizations can help users identify patterns and connections between clusters more easily. However, it is important to note that these visualizations are not always clear or pertinent to the product’s subject matter, and users may need additional context or explanations to fully understand them.
Overall, both High-MAST and Low-MAST have limitations in terms of transparency, accuracy, and clarity of their results. While High-MAST provides more detailed information about the data used to derive the clusters, it does not offer a complete picture of how the titles or summaries are calculated. Low-MAST, on the other hand, offers more details about a given cluster but lacks transparency in its methodology and results. Therefore, it is essential to carefully evaluate these systems and consider their limitations when using them for analysis or decision-making purposes.
Computer Science, Computers and Society
Assessing the Accuracy of AI-Driven Document Clustering: A Comparative Study
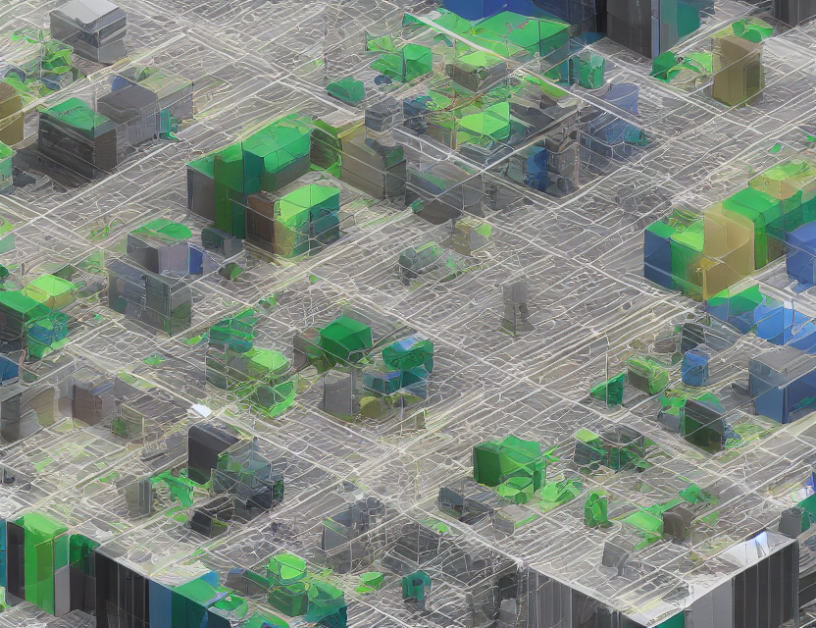