The article discusses the development and evaluation of a benchmark for multimodal language models (MLMs) called Prompt Suite. The authors aim to provide a standardized platform for evaluating MLMs across different content categories, such as Animal, Architecture, Food, Human, Lifestyle, Plant, Scenery, and Vehicles.
To create the benchmark, the authors first use a large language model (LLM) to categorize a collection of human-curated prompts into eight content categories. They then select 800 prompts from each category and manually clean their labels to serve as per-category prompt suites. Finally, they obtain 100 prompts for each of the eight categories.
The authors evaluate the performance of their Prompt Suite across varied content types using a variety of evaluation dimensions, including lexical, syntax, semantics, and pragmatics. They show that their benchmark provides a comprehensive and accurate assessment of MLMs’ performance across different content categories.
The authors also introduce an interface for human preference annotation, which allows annotators to provide fine-grained judgments on the quality of the generated responses. This interface is designed to capture the nuances of natural language understanding and generation, and to provide a more comprehensive evaluation of MLMs’ performance.
In summary, the authors have developed a benchmark for evaluating MLMs across different content categories, called Prompt Suite. The benchmark provides a standardized platform for evaluating MLMs’ performance and offers a more comprehensive assessment of their abilities in understanding and generating natural language.
Computer Science, Computer Vision and Pattern Recognition
Comparing Different Approaches to Text-Driven Video Editing
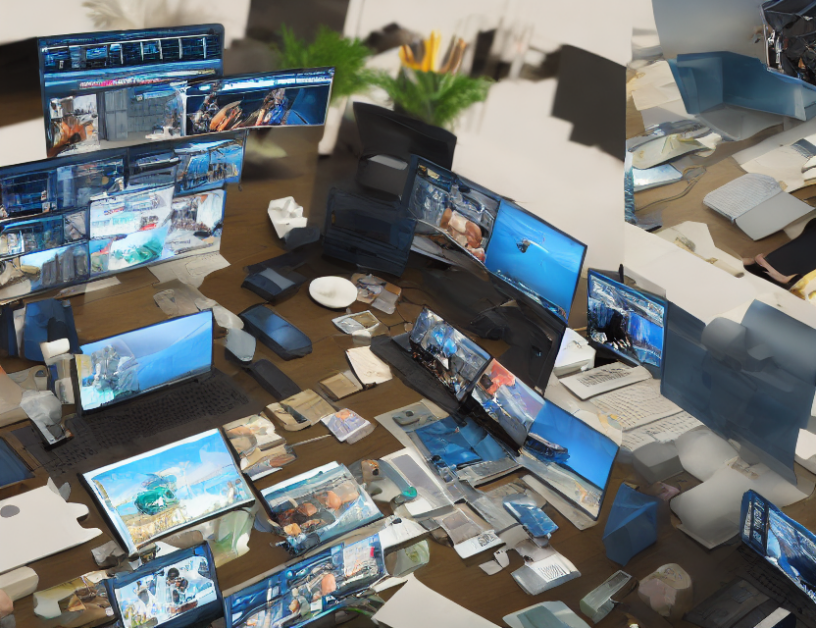