In this article, the authors propose a new approach to Referring Image Search (RIS) called Bidirectional Transformer-based Multi-modal Attention Enhancement (BTMAE). RIS is a challenging task as it involves matching visual features in an image with the textual descriptions of objects or scenes in the image. Traditional RIS models rely on Multi-modal Attention (MAE) to capture multi-modal context information, but they suffer from limitations such as ignoring spatial information and producing irrelevant attention weights.
BTMAE addresses these limitations by incorporating both spatial and non-spatial attention mechanisms into the transformer-based encoder. The proposed model uses a novel bidirectional transformer architecture that captures both local and global contextual information in the image and text modalities. This allows the model to effectively enhance the performance of RIS even with a small dataset.
Experiments conducted on three commonly used datasets show that BTMAE achieves state-of-the-art performance compared to other RIS models. The authors also demonstrate the effectiveness of their approach through various ablation studies, showing that it can produce robust RIS results in challenging scenes.
In summary, BTMAE is a novel approach to RIS that leverages both spatial and non-spatial attention mechanisms to improve the performance of the model. By incorporating these mechanisms into a transformer-based encoder, the proposed model can effectively capture multi-modal context information and enhance the performance of RIS even with a small dataset.
Computer Science, Computer Vision and Pattern Recognition
Enhancing Referring Image Segmentation with Multi-Modal Context
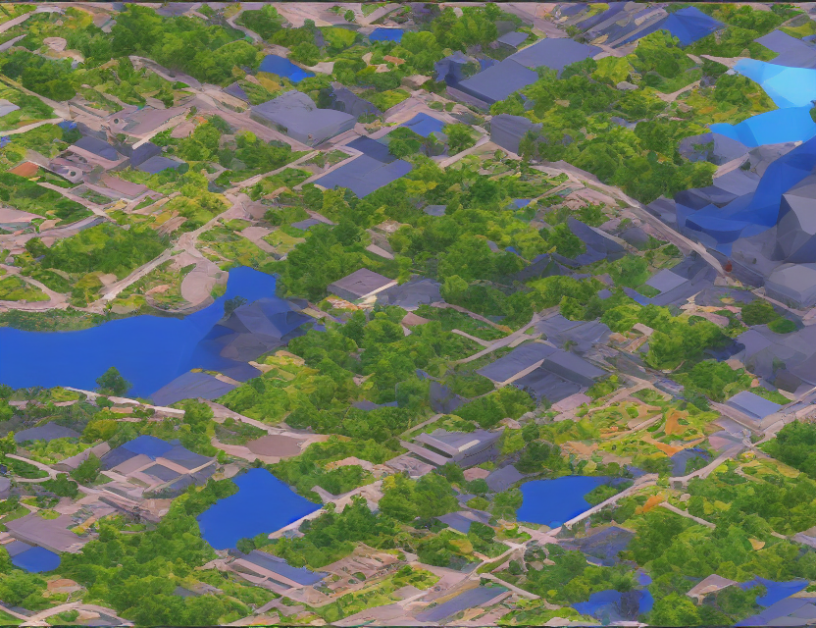