In this article, we investigate the significance of textual information in vision-and-language models (VLMs) for image retrieval tasks. VLMs are trained to generate answers to questions based on visual content and textual context. We explore how different textual settings affect the performance of these models and find that including textual information can significantly improve their ability to recall relevant demonstrations, even when the visual content is the same.
To evaluate the importance of textual information, we create various demonstration settings where the image-question pairs are either randomly selected or replaced with different answers for the same question. We observe that while the visual content plays a crucial role in retrieving demonstrations, textual information acts as a key factor in achieving better performance. In particular, when the textual context is rich and informative, VLMs can better understand the query and retrieve more relevant demonstrations.
Our findings highlight the significance of incorporating textual information into VLMs for image retrieval tasks. By using textual cues, these models can better comprehend the context and generate more accurate responses to queries. In essence, textual information serves as a guide that helps VLMs navigate through the complex landscape of visual content and retrieve relevant demonstrations with greater accuracy.
In conclusion, our study underscores the importance of textual information in VLMs for image retrieval tasks. By incorporating textual cues into these models, we can improve their ability to recall relevant demonstrations and provide more accurate responses to queries. This finding has significant implications for applications where visual content is abundant but the contextual information is limited, such as in robotics or autonomous vehicles.
Computer Science, Computer Vision and Pattern Recognition
Enhancing Vision-Language Models with Multi-Modal In-Context Learning
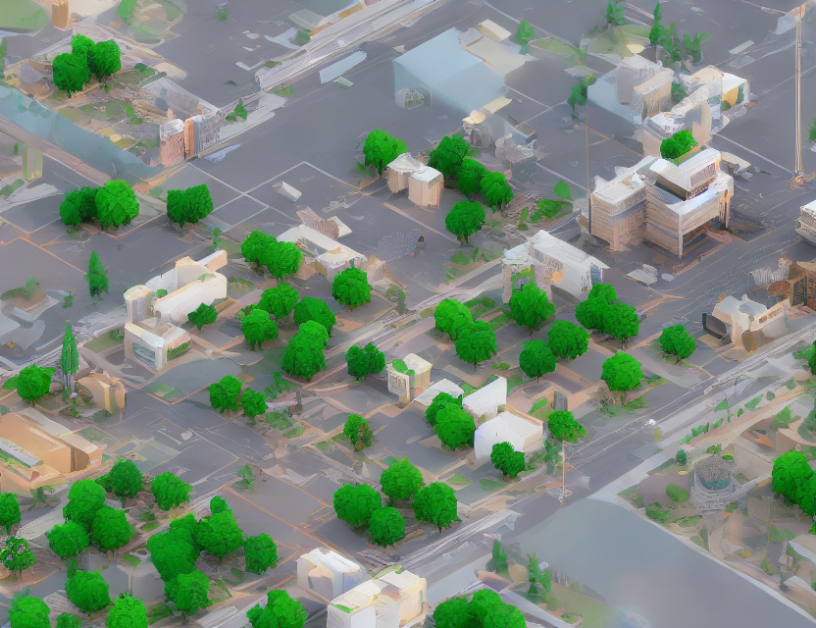