In this article, the authors explore the effectiveness of attention-based modulation in improving scene text image super-resolution (STISR). They propose a novel module called Attention-based Modulation Module (AMM), which captures long-range dependence and restores visual structure to images with deformed or long text. The AMM is designed with multiple blocks, each consisting of a convolutional layer, local attention module, and global attention module. The authors demonstrate the superiority of their proposed method over existing techniques in terms of both objective metrics and visual quality.
The article begins by introducing the problem of STISR and the importance of capturing long-range dependence to improve image quality. The authors then provide a comprehensive review of existing methods, including diffusion models, which have shown promising results in this area. They highlight the limitations of these methods, such as their reliance on heuristics and the lack of semantic guidance during the super-resolution process.
To address these limitations, the authors propose the AMM, which leverages attention mechanisms to provide the SR network with enhanced semantic guidance. The AMM is designed to capture long-range dependence by incorporating both local and global attention, allowing it to effectively restore visual structure in images with deformed or long text.
The authors evaluate their proposed method on several benchmark datasets and compare it to existing techniques. They show that the AMM significantly outperforms these methods in terms of both objective metrics and visual quality, demonstrating its effectiveness in improving STISR. Additionally, they provide a qualitative analysis of the generated images, highlighting the improved semantic accuracy and visual structure.
In conclusion, this article provides a novel approach to STISR by leveraging attention-based modulation. The proposed AMM effectively captures long-range dependence, resulting in images with enhanced semantic accuracy and visual structure. The authors demonstrate the superiority of their method over existing techniques, making it a promising solution for improving scene text image super-resolution.
Computer Science, Computer Vision and Pattern Recognition
Hierarchical Vision Transformer Using Shifted Windows
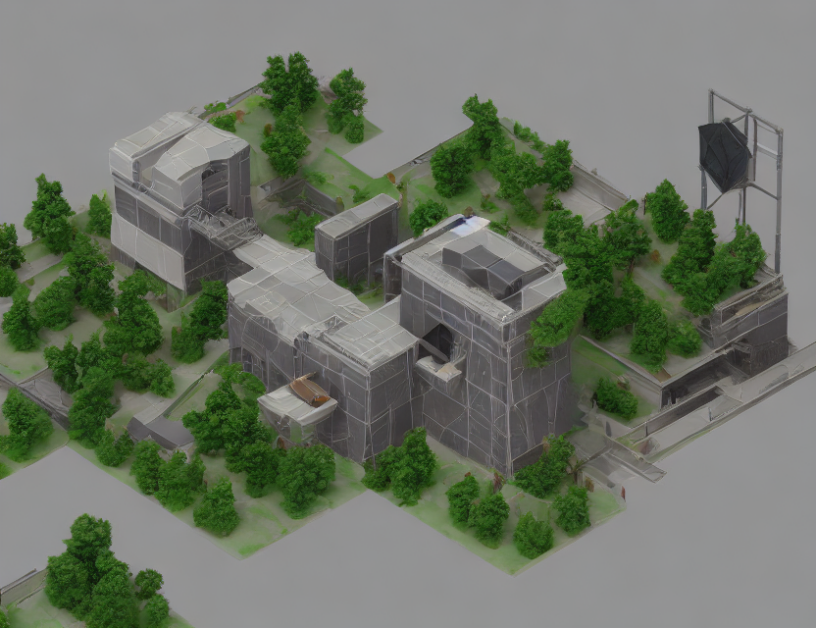