Systematic Literature Review (SLR): A Comprehensive Approach to Understanding Non-Functional Requirements of Machine Learning Enabled Systems
In this article, we present a systematic literature review (SLR) that aimed to identify and analyze existing scientific papers on non-functional requirements of machine learning (ML) enabled systems. The SLR was designed to address the research question: "What are the non-functional requirements of ML-enabled systems?"
The authors began by following established guidelines proposed by Kitchenham et al. [42], which provided a framework for conducting an SLR. However, they had to make adjustments to ensure the sustainability of the data collection and analysis procedures when designing the search string. The search string was designed to identify articles that explicitly referred to terms like "Non-Functional Requirements" and "Machine Learning."
The authors collected six main categories of information through their SLR, including publication year, venues, dimension, description, and specific attributes. They used this information to statistically describe their sample in terms of bibliometrics and to describe the trends in terms of publication, the most relevant venues accepting research papers on non-functional requirements of ML-enabled systems.
The authors emphasize that their SLR aimed to provide a comprehensive overview of the existing literature on non-functional requirements of ML-enabled systems. They note that the study’s findings highlight the importance of considering non-functional requirements in the development and deployment of ML-enabled systems.
In summary, this article presents a systematic literature review that aimed to identify and analyze existing scientific papers on non-functional requirements of machine learning enabled systems. The authors used established guidelines for conducting an SLR and made adjustments to ensure the sustainability of their data collection and analysis procedures. They collected six main categories of information and used this information to statistically describe their sample and describe trends in publication. The study highlights the importance of considering non-functional requirements in the development and deployment of ML-enabled systems.
Computer Science, Software Engineering
Missed Papers in Search String: Impact on Addressing Research Questions
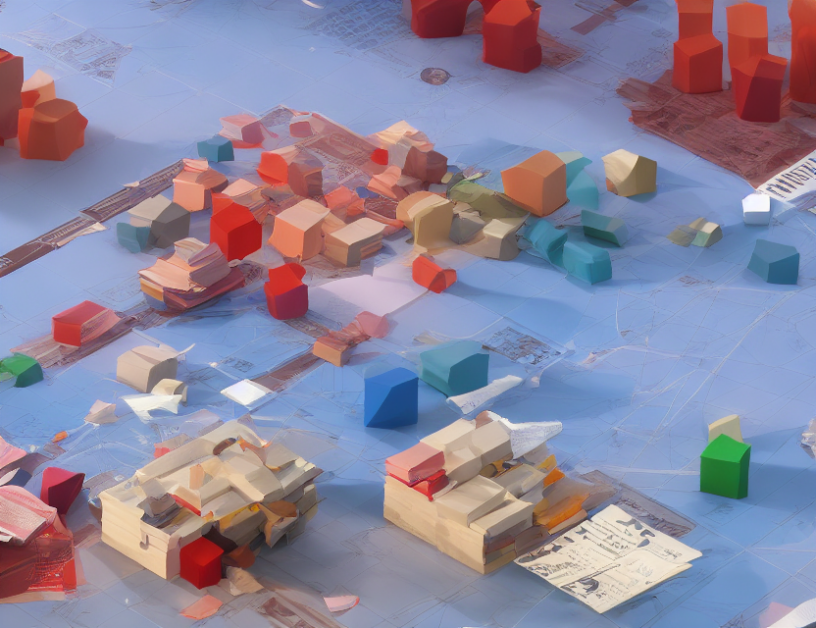