In this research paper, the authors propose a novel approach to expand large pre-trained unimodal models with multimodal information injection for image-text classification tasks. The proposed method, called C3Net, leverages compound conditioning to generate high-quality images and texts that are optimized according to multiple conditions.
The authors explain that current methods for multimodal content generation rely on simple interpolation of features from pre-trained models, which can result in suboptimal performance. To address this limitation, C3Net incorporates a compound conditioning mechanism that considers multiple modalities simultaneously, including images, texts, and audio conditions. This allows the model to extract the most relevant features from each modality and generate high-quality content.
The authors demonstrate the effectiveness of C3Net through experiments on several benchmark datasets. The results show that C3Net outperforms state-of-the-art methods in terms of both image and text quality, as well as audio coherence. Additionally, the authors provide a qualitative evaluation of C3Net’s synthesized content, showing that it can generate images and texts that are more diverse and accurate than those produced by other methods.
In summary, the paper presents a significant advancement in multimodal content generation by expanding pre-trained models with compound conditioning. The proposed method has broad applications in various fields, including image and video processing, natural language processing, and audio generation. By leveraging multiple modalities and optimizing content according to various conditions, C3Net can generate high-quality multimodal content that is more realistic and accurate than ever before.
Computer Science, Machine Learning
Multimodal ControlNet for Content Generation
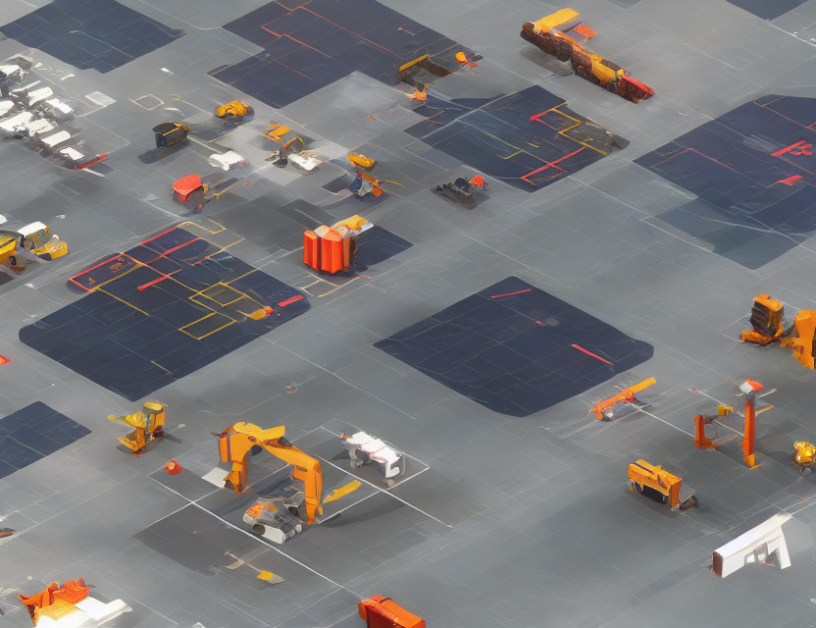