Counting and Quantification in Data Annotation
Data annotation is a crucial process in machine learning, where humans label data to help AI systems learn and improve. However, the process of counting and quantifying this work has significant implications for the workers involved and the AI supply chain as a whole.
The article argues that counting and quantification reduce complex social realities into unitary tasks that can only be known and rendered as numbers. This reduction of complexity poses the risk of normalizing single figures, which can overlook important contextual complexities. For example, standard costing and budgeting in the early 20th century helped to manage workers more efficiently, but these practices also contributed to notions of individual efficiency being expressed in monetary terms. Similarly, today’s adoption of data-intensive technologies in various domains has led to the quantitation of work, which can inform editorial decisions and shape journalistic practice.
The article highlights two main concerns with counting and quantification: epistemological and practical. Firstly, counting produces a "realization" of theoretical categories rather than a "representation" of the context, which can lead to a loss of nuance in understanding complex phenomena. Secondly, the framing of numerical enquiries, systems of classification, methods of measurement, and frequency of measurement all embody and embed the expectations, beliefs, and concerns of those responsible for counting.
To demystify these complex concepts, the article uses analogies such as a recipe to explain how counting works. Just as a recipe breaks down a complex process into simpler steps, counting reduces complex social realities into unitary tasks that can be measured and analyzed. However, just as a recipe cannot capture the full flavor and texture of a dish, counting cannot fully capture the complexity of what it is counting.
In conclusion, the article emphasizes the importance of considering the role of counting as a structural activity in the workplace, involving actors, practices, and technologies. By recognizing the limitations of counting and its implications for workers and the AI supply chain, we can develop a more nuanced understanding of the complexities of data annotation and the role it plays in shaping our understanding of the world around us.
Computer Science, Human-Computer Interaction
Power Dynamics in Data Annotation: A Critical Examination of Bias and Control
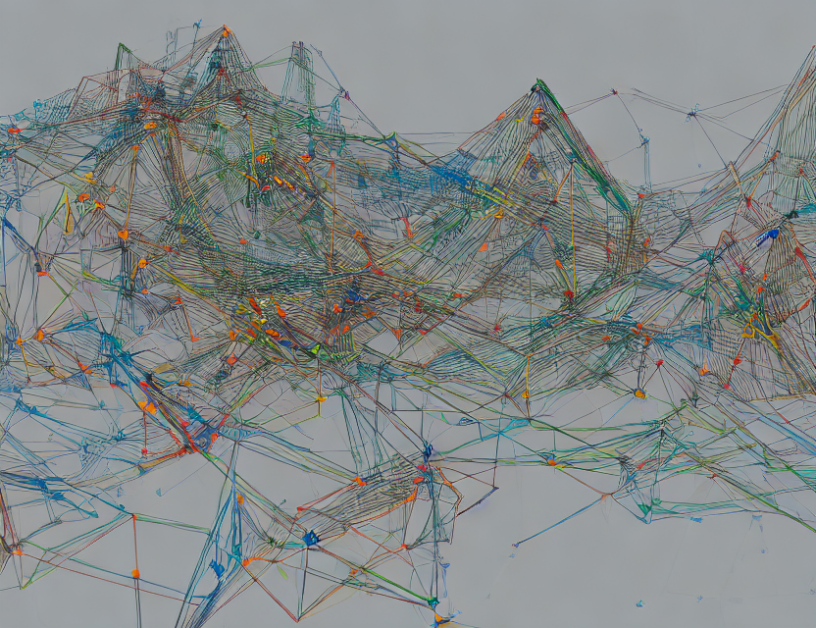