In this study, researchers aimed to develop data-driven models for energy consumption prediction in commercial buildings. They used a dataset of 500 buildings and applied various machine learning algorithms, including Random Forest and Gradient Boosting, to evaluate their performance. The findings showed that the combination of domain-informed features and off-the-shelf solutions can lead to accurate predictions.
To begin with, the researchers explained the importance of energy consumption prediction in commercial buildings, highlighting its potential for cost savings and environmental benefits. They then described their dataset, which included time series data on electricity consumption from 500 commercial buildings in California.
Next, the authors discussed their approach to developing data-driven models for energy consumption prediction. They explained that they used a combination of domain-informed features and off-the-shelf solutions to improve the accuracy of their predictions. They also described how they employed techniques such as feature importance analysis to identify the most critical features for their models.
The researchers then presented their findings, which showed that their combined model achieved an RMSE value of 19.3% and an MAE value of 12.8%. They noted that four out of the top five features in their combination model were domain-informed features, indicating the effectiveness of their approach.
Finally, the authors discussed the implications of their findings and suggested future areas of research. They highlighted the potential for further improving energy consumption predictions by incorporating additional data sources and refining their machine learning algorithms.
In conclusion, this study demonstrates the feasibility of developing accurate data-driven models for energy consumption prediction in commercial buildings using a combination of domain-informed features and off-the-shelf solutions. The findings have important implications for building owners and managers seeking to reduce energy costs and minimize their environmental impact. By leveraging machine learning algorithms and feature importance analysis, researchers can improve the accuracy of their predictions and support sustainable practices in the built environment.
Computer Science, Machine Learning
Predicting Hourly Building Electricity Consumption Using Historical and Forecast Weather Data
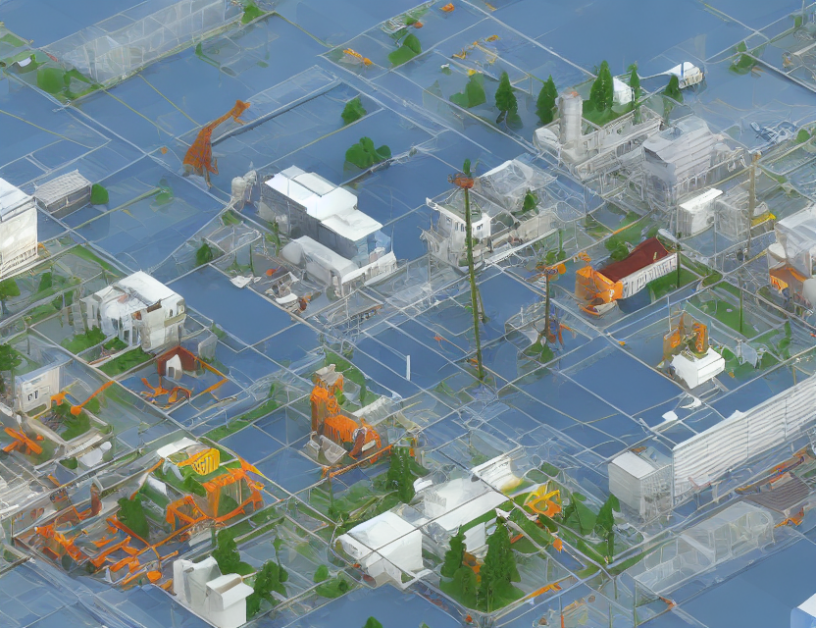