In this article, the authors propose a novel method for active learning in hyperspectral image classification called view generation. Active learning is a technique that involves actively selecting the most informative samples to classify, rather than randomly sampling the entire dataset. The authors’ approach leverages multiple views or features of the images to improve the accuracy of the selected samples and reduce the number of misclassifications.
The authors introduce two pretext tasks: (1) semantic segmentation and (2) image denoising. These tasks are designed to learn different representations of the images, which can be used as views for active learning. The authors then propose a method to combine these views into a single representation that captures the underlying patterns in the data.
The proposed method is evaluated on a real-world hyperspectral image dataset, and the results show improved accuracy compared to traditional random sampling methods. The authors also demonstrate the effectiveness of their approach in scenarios where labeled data is scarce or difficult to obtain.
In summary, this article presents a novel active learning method for hyperspectral image classification that leverages multiple views or features of the images to improve the accuracy of the selected samples. By combining semantic segmentation and image denoising as pretext tasks, the authors are able to learn more robust representations of the data, which can be used to improve the performance of the classification model. The proposed method has important implications for applications where labeled data is limited or expensive to obtain, such as environmental monitoring, agricultural management, and military surveillance.
Computer Science, Computer Vision and Pattern Recognition
Semi-Supervised Learning Techniques for Improved Image Classification
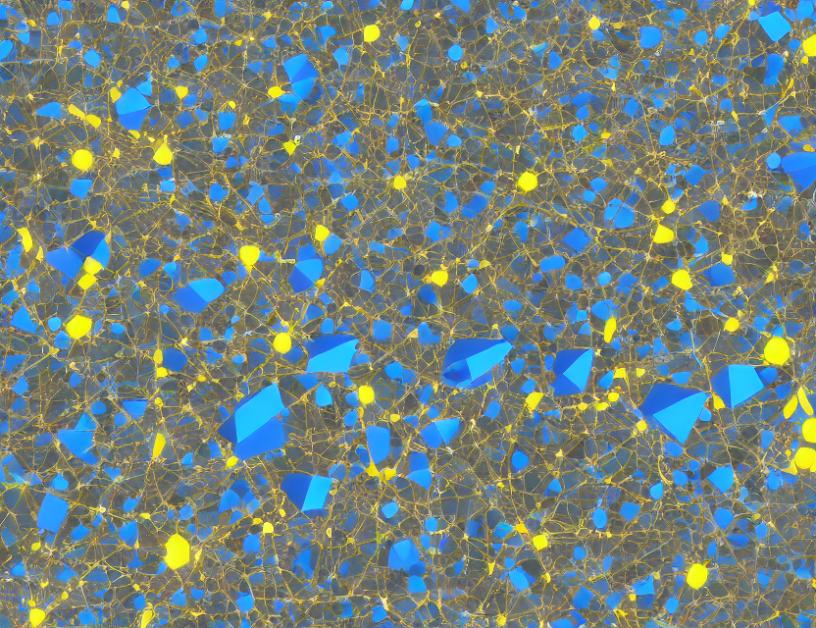