In this article, the authors propose a novel approach to unify localization and vision-language understanding (VLU) called GLIP V2. The main goal is to improve the accuracy of VLU models by incorporating localization information. The authors argue that previous methods have focused solely on the visual features of images, neglecting the importance of language context.
To address this issue, GLIP V2 combines both generative and contrastive loss functions. The generative loss encourages the model to generate accurate captions for an image, while the contrastive loss ensures that the visual features are aligned with the corresponding word embeddings. By combining these two losses, the model can learn to attend to subtle visual features that may not be apparent from just looking at the image alone.
The authors evaluate GLIP V2 on several benchmark datasets and show that it outperforms previous state-of-the-art models. They also demonstrate the effectiveness of their approach by showing that GLIP V2 can localize objects in images more accurately than previous methods.
In summary, GLIP V2 is a novel approach to unifying localization and VLU that combines both generative and contrastive loss functions. By attending to subtle visual features, the model can improve the accuracy of VLU models and provide better localization results. This work has important implications for applications such as robotics, autonomous driving, and medical imaging, where accurate visual understanding is crucial.
Computer Science, Computer Vision and Pattern Recognition
Visual Instruction Tuning for Image Captioning with Embedded Word Embeddings
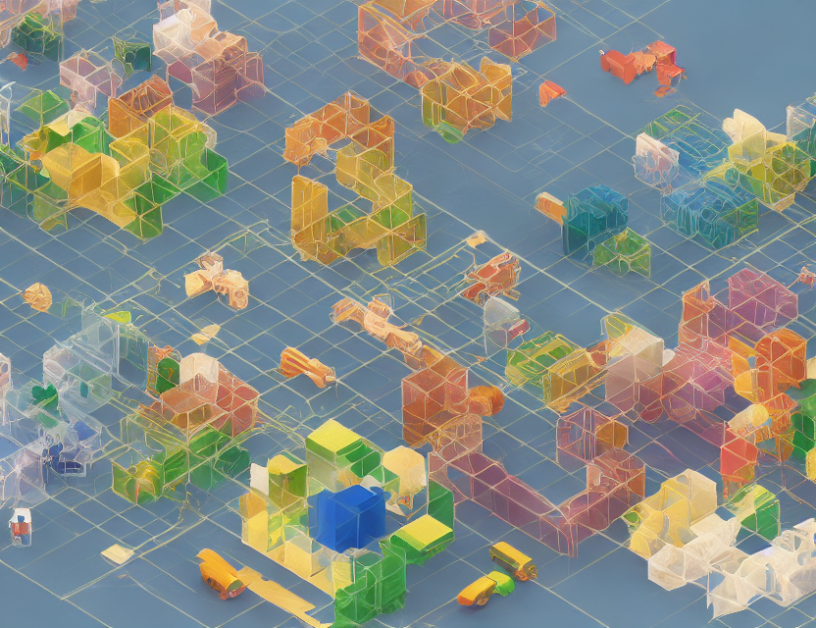