In this section, the authors present their approach to synthesizing colonoscopic data using Blender, a 3D modeling and rendering software suite. They explain how they generated a colon-like deforming surface with freeform deformation, represented by a triangular mesh with 502,502 vertices and 1,002,000 faces, over 250 frames. The surface was imparted with a strong "clearcoat" to boost specular reflections, mimicking real-world shading and specularities using the anisotropic principled BSDF model [10].
To create the data set, the authors used the origin at the principal point and measured distances in units of focal length. They also referenced relevant works in computer graphics and image processing to provide context for their approach.
Understanding this section is crucial because it lays the foundation for the subsequent sections, which focus on analyzing the synthetic colonoscopic data set created by the authors. By familiarizing ourselves with their methodology, we can better appreciate how they validate their approach and demonstrate its effectiveness in simulating real-world scenarios.
To simplify this complex concept, let’s consider it like building a virtual Lego model of a colon. Just as architects use software to create detailed 3D models of buildings before constructing them, the authors employ Blender to craft a lifelike representation of a colon’s internal structures. They then manipulate this digital model in various ways to simulate realistic deformations and movements, mimicking what endoscopists might observe during a procedure.
In summary, the authors’ approach involves generating synthetic colonoscopic data using Blender, creating a detailed 3D mesh of a colon with freeform deformation, and imparting it with realistic shading and specularities to mimic real-world conditions. By understanding this process, we can better appreciate their subsequent analyses and evaluations of this data set, which are crucial for advancing the field of endoscopy.
Computer Science, Computer Vision and Pattern Recognition
Advances in Endoscopic Depth Prediction Using Conditional Generative Adversarial Networks
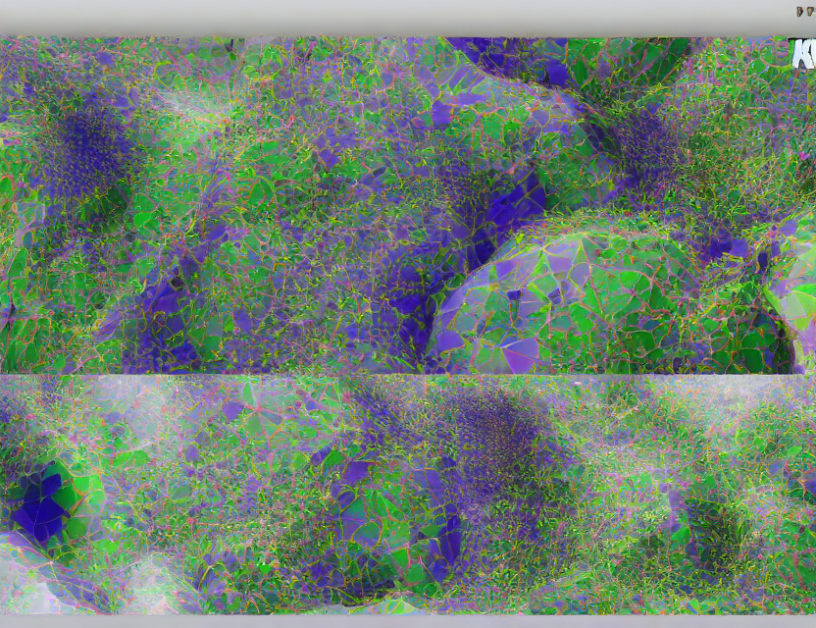