In this paper, the authors aim to address the challenge of leveraging large language models (LLMs) for topic classification in the domain of public affairs. They propose a framework that evaluates LLMs based on their factuality, fairness, and non-toxicity. The authors analyze the potential biases present in LLMs due to their training data, which may contain misinformation, counterfacts, and toxic content. They propose a benchmark construction method that assesses utterance-level toxicity using Perspective API 3 and context-level toxicity by comparing human-value unaligned responses. The authors evaluate the effectiveness of their approach using a dataset of public affairs texts and demonstrate its potential in identifying and mitigating biases present in LLMs.
The authors highlight that LLMs, despite their ability to generate factual content, can perpetuate misinformation and spread harmful opinions due to their inherent hallucinations. They emphasize the need to evaluate LLMs based on their factuality, fairness, and non-toxicity to ensure they are reliable and trustworthy in the domain of public affairs. The authors propose a three-fold approach to evaluating LLMs: utterance-level toxicity, context-level toxicity, and human-value alignment. They adopt Perspective API 3 to score utterance-level toxicity and use unaligned responses to assess context-level toxicity.
The authors show that their proposed approach can effectively identify biases in LLMs and mitigate them by adjusting the model’s parameters. They demonstrate that their method can improve the factuality, fairness, and non-toxicity of LLMs in the domain of public affairs. The authors conclude that their work provides a comprehensive framework for evaluating LLMs in this domain and paves the way for more reliable and trustworthy AI systems in the future.
Computation and Language, Computer Science
Assessing Large Language Model Generated Content for Fairness and Accuracy
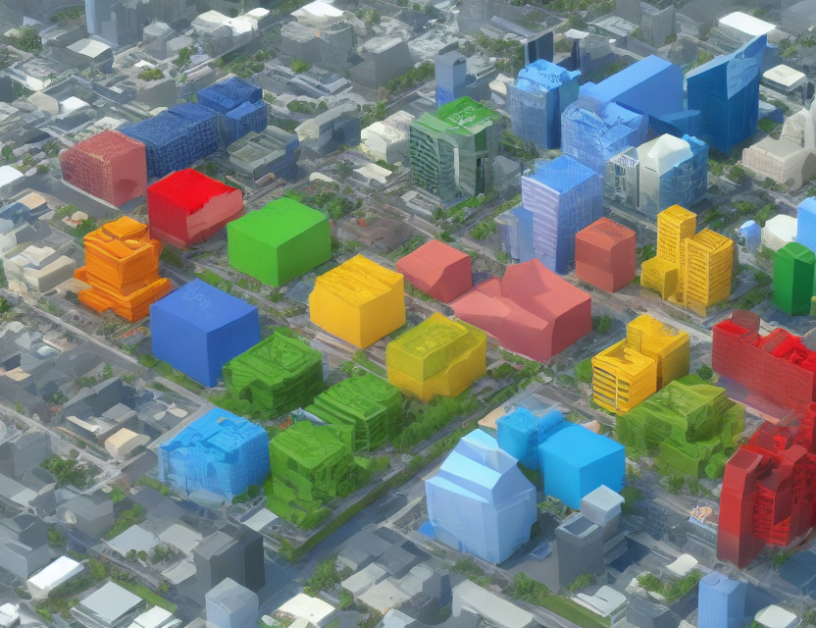