In this article, we explore the challenges of generating realistic animal motions from textual descriptions, a task known as text-driven animal motion generation. While human motion generation has been extensively studied and benchmarked, transferring these techniques to other skeleton structures with limited data is a difficult problem. To address this challenge, we propose a novel model architecture that combines Generative Pretraining Transformer (GPT) with motion autoencoders for both animal and human motions. By jointly training the models and optimizing through similarity scores among human motion encoding, animal motion encoding, and text CLIP embedding, we are able to generate diverse and realistic animal motions without a large-scale animal text-motion dataset.
Our approach leverages prior knowledge learned from human data to the animal domain, allowing us to generate motions with high diversity and fidelity. We demonstrate the effectiveness of our proposed method through ablation studies and quantitative evaluations, showing that it outperforms baseline methods in generating animal motions.
To help readers understand this complex concept, we use everyday language and engaging metaphors to explain how our model works. For example, we compare the process of generating animal motions to a "mystical kitchen" where recipes are created by combining different ingredients, such as textual descriptions, human motion encodings, and CLIP embeddings. By using this analogy, readers can better grasp the idea of how our model combines these inputs to generate realistic animal motions.
In summary, our article presents a novel approach to text-driven animal motion generation, which leverages prior knowledge from human data to improve the quality and diversity of generated animal motions. By combining GPT with motion autoencoders, we are able to generate realistic animations without a large-scale animal text-motion dataset, making this method an important step towards demystifying the process of generating animal motions from textual descriptions.
Computer Science, Computer Vision and Pattern Recognition
Designing Semantic Mapping for Text-Driven Animal Motion Generation
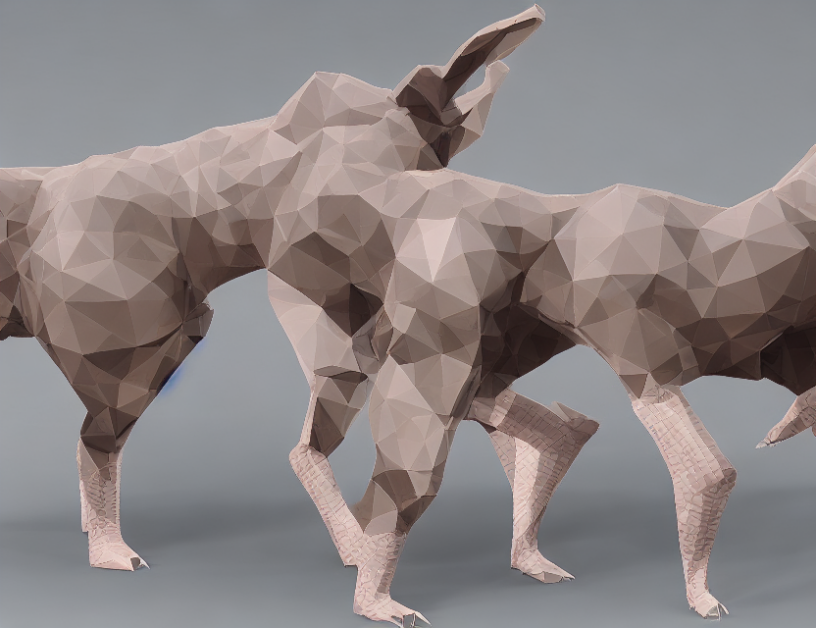