In this study, researchers aimed to improve the accuracy of precipitation nowcasting (predicting rainfall in the short term) using a new architecture called SmaAt U-Net. The U-Net is a type of neural network that has been successful in image recognition tasks. The SmaAt U-Net model uses a small attention mechanism, which helps the network focus on important parts of the input data, to improve its performance.
The researchers experimented with different variations of the SmaAt U-Net architecture and found that their best model outperformed the baseline model, which is commonly used in precipitation nowcasting. However, despite this improvement, the new model still falls short of achieving substantial gains in accuracy.
To put this into perspective, the researchers pointed out that if we consider the number of parameters in a model as a proxy for its computational demand, improving the score of the baseline model by a fraction of its computational cost is an achievement in itself. Therefore, while the new model shows improvement, it still has room for further advancements.
The study highlights the ongoing challenge in balancing model performance and energy efficiency, particularly as the field of deep learning continues to evolve towards larger and more complex architectures. The researchers argue that it is essential to evaluate models based on both their accuracy and environmental impact, and they advocate for a holistic approach to evaluating model efficacy.
In summary, the SmaAt U-Net architecture shows promise in improving precipitation nowcasting accuracy, but there is still room for improvement in terms of balancing performance and energy efficiency. The study underscores the need for a comprehensive evaluation framework that considers both the accuracy of models and their environmental impact.
Computer Science, Machine Learning
Enhancing Energy Efficiency in Deep Learning for Weather Forecasting
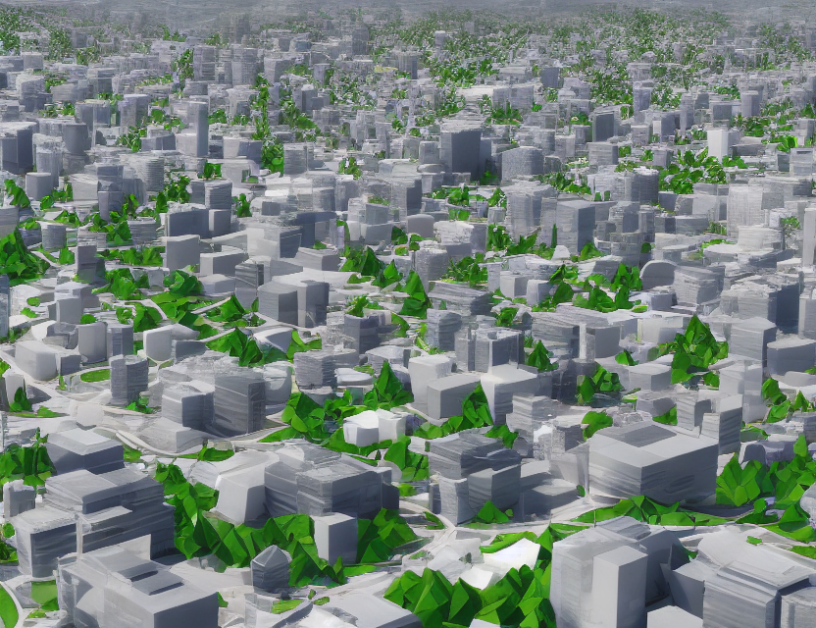