In this article, the authors propose a novel approach to open-vocabulary scene understanding, which enables a deep learning model to accurately recognize objects and their properties in complex scenes from different viewpoints. The proposed method leverages adaptive semantic smoothing, a technique that selectively applies spatial consistency to manage variance and ambiguity in the model’s predictions.
To better understand this concept, imagine you’re trying to find specific objects in a busy and ever-changing environment like a toy store. The objects are scattered all over the place, and some of them might be partially hidden or obscured from view. That’s where our deep learning model comes in – it’s like a highly trained eye that can quickly identify each object and understand its properties, even when they’re seen from different angles or lighting conditions.
The key innovation of the proposed method is the use of adaptive semantic smoothing, which is like a special kind of filter that only applies to areas where the model needs more help in understanding. This filter ensures that the model can confidently recognize objects and their properties even when they’re seen from unusual viewpoints or have complex shapes.
To put it simply, our method enables a deep learning model to understand scenes like a human would, by adapting to different viewpoints and managing ambiguity in real-time. This makes it possible for the model to accurately recognize objects and their properties, even when they’re partially hidden or seen from unusual angles.
In summary, this article proposes an innovative approach to open-vocabulary scene understanding that leverages adaptive semantic smoothing to manage variance and ambiguity in deep learning models. By adapting to different viewpoints and selectively applying spatial consistency, the proposed method enables accurate object recognition and property prediction even in complex and dynamic scenes.
Computer Science, Computer Vision and Pattern Recognition
Improved Semantic Segmentation for Open-Vocabulary Queries Using NeRF and Panoptic Neural Fields
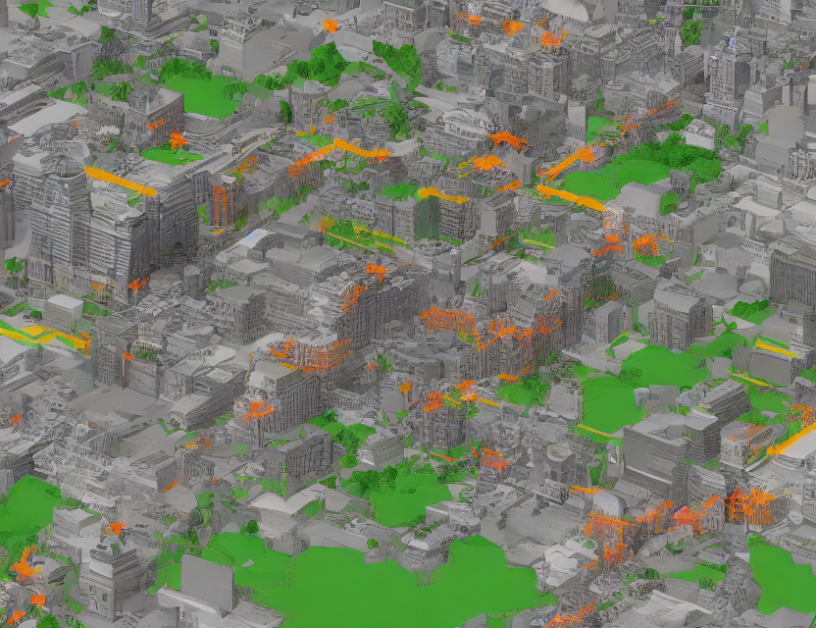