In this paper, researchers propose a novel communication framework that enables two-intelligent agents to share information effectively and efficiently. The proposed framework leverages the emerging concept of "theory of mind" (ToM) in machine learning to facilitate goal-oriented information sharing. To achieve this, the authors introduce a two-level feedback mechanism – one wirelessly transmitted and another semantically interpreted at the receiver’s end.
At the higher level, the authors focus on defining semantics as the causal state that captures the fundamental relationships and dependencies among various features extracted from data. The transmitter uses this semantic information to fine-tune its neural network components, mimicking the receiver’s mental state. In turn, the receiver dynamically updates its estimated mental state through the same two-level feedback mechanism.
To simplify complex concepts, imagine a conversation between two people, where one person (the transmitter) tries to convey meaningful information to the other person (the receiver). Semantics here represents the causal link between the words used and their intended meanings. The proposed framework acts as a mediator, ensuring that both parties share the same understanding of the conversation’s context.
The authors emphasize that their approach is designed to handle complex scenarios where agents have different goals, beliefs, and perspectives. By leveraging ToM, the transmitter can adapt its communication strategy based on the receiver’s mental state, making the exchange more effective and efficient.
In summary, the proposed framework provides a novel approach to pragmatic semantic communication that enables two-intelligent agents to share information goal-oriented manner. By leveraging ToM, the authors create a dynamic two-level feedback mechanism that continuously updates both parties’ mental states, leading to more effective and efficient communication.
Computer Science, Information Theory
Learning to Communicate: Semantic Systems for Improved Real-Time Decision Making
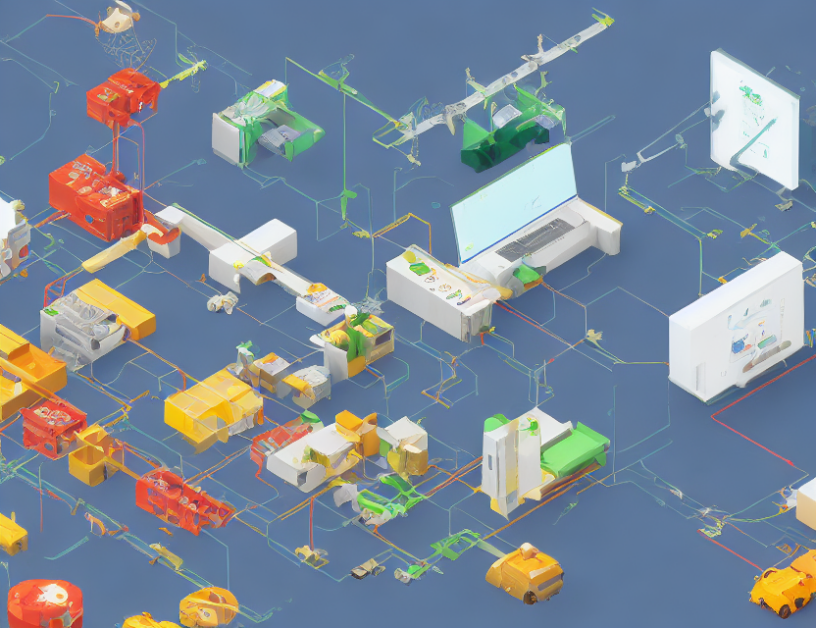