In this article, researchers propose a novel method called "Dense Keypoint-Box Text-to-Image Diffusion" to generate high-quality images from detailed text descriptions. The approach involves extending existing keypoint-based diffusion models to incorporate attention modulation and ground diverse attributes in the generated images. This allows for meticulous control over human instances, including factors like gender, clothing color, and character attributes.
The proposed method is evaluated through user studies and comparison with state-of-the-art methods. The results show that our DetText2Scene enabled large-scene synthesis from detailed human-centric text with exquisite quality, achieving firm faithfulness, strong controllability, and high naturalness across various aspects. The ablation studies demonstrate that our method outperforms prior arts in text-to-scene generation even when keypoint-box layouts are given.
To understand this concept, imagine a magician who can conjure up any image from a detailed description. Just like how a magician uses different tricks to create illusions, the researchers’ method uses attention modulation and grounding diverse attributes to generate images that are faithful to the text descriptions. By incorporating these elements, the proposed method achieves unparalleled control over the generated images, allowing for meticulous manipulation of human instances.
In summary, the article introduces a novel approach called Dense Keypoint-Box Text-to-Image Diffusion that enables large-scene synthesis from detailed text descriptions with unprecedented quality and control. The proposed method leverages attention modulation and grounding diverse attributes to create images that are faithful to the text, making it an exciting breakthrough in the field of computer vision and natural language processing.
Computer Science, Computer Vision and Pattern Recognition
Personalizing Text-to-Image Generation with Hierarchical Keypoint-Box Layout
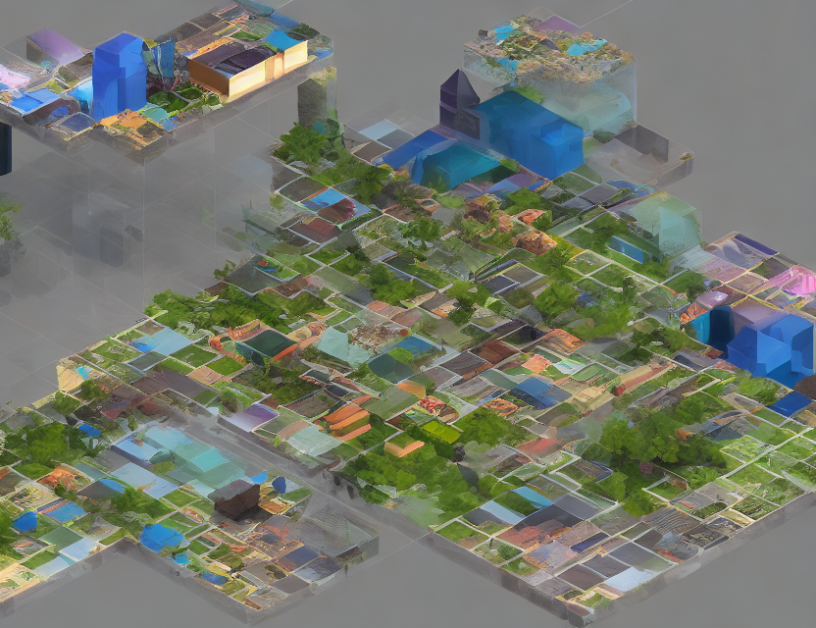