Autonomous driving technology aims to make roads safer by enabling vehicles to navigate without human input. However, developing this technology requires vast amounts of data and computing power to train machine learning models that can mimic human driving decisions. Mars is a novel solution that addresses these challenges by creating an "instant-aware," modular, and realistic simulator for autonomous driving.
The authors propose a new approach called instance-aware rendering, which allows the simulator to generate diverse and complex scenarios, such as pedestrians or other vehicles, in a way that is similar to how they would appear in real life. This is achieved by using a multi-layer perception network that can handle various instances of objects in a scene, rather than simply generating predefined templates.
Mars also employs a modular architecture, which enables the simulator to be easily extended or modified to accommodate different driving scenarios and environments. This allows researchers to focus on specific aspects of autonomous driving without having to rebuild the entire system.
The authors demonstrate the effectiveness of Mars through extensive experiments using real-world data from KITTI, a benchmark dataset for autonomous driving research. They show that Mars can generate high-quality novel views of scenes similar to those in KITTI, and that these views are indistinguishable from real-world footage.
In summary, Mars is a powerful tool for simulating autonomous driving scenarios that is both efficient and flexible. By using instance-aware rendering and modular architecture, Mars can generate highly realistic scenes that mimic real-world driving conditions, allowing researchers to test and develop their algorithms more efficiently and accurately than ever before.
Computer Science, Computer Vision and Pattern Recognition
Revolutionizing Autonomous Driving with Novel View Synthesis
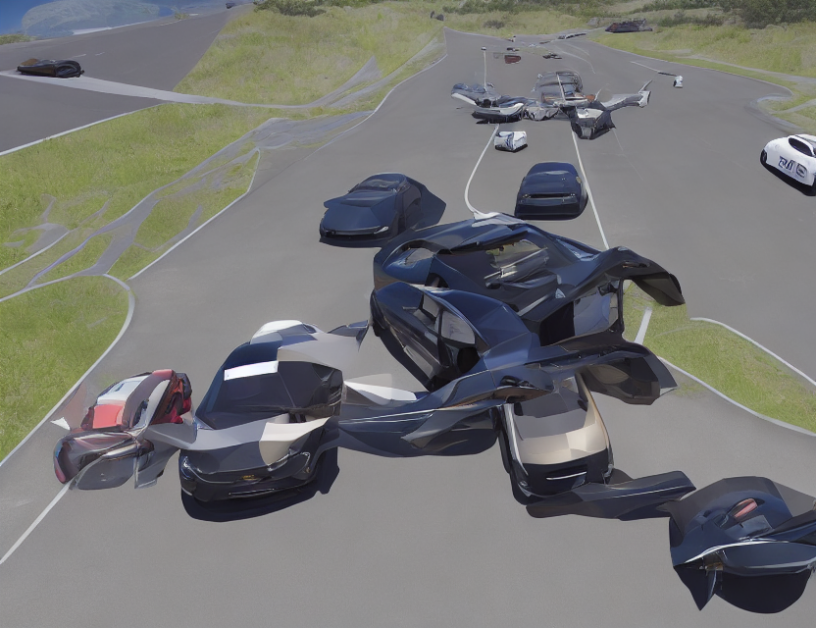