In this article, we propose a new method called ZEST-NERF to improve the efficiency and flexibility of Neural Radiance Fields (NeRF) for dynamic scenes. NeRF is a popular approach that represents scenes as neural networks, allowing for photo-realistic rendering and novel view synthesis. However, current NeRF methods have limitations, such as requiring a lot of input views, being expensive, and having a long optimisation process per scene.
To address these challenges, ZEST-NERF leverages the temporal dimension alongside the spatial ones. This allows for more efficient and flexible rendering of dynamic scenes, with fewer input views and reduced computational cost. We achieve this by using a multiresolution hash encoding and a hierarchical representation of the scene, which enables the model to focus on the most important information and ignore the less relevant details.
Our approach can be thought of as a kind of "Scene-Aware" NeRF, as it takes into account the temporal context of the scene, allowing for more accurate and efficient rendering of dynamic scenes. This is particularly useful in applications such as virtual reality, where realistic and efficient rendering of dynamic scenes is crucial.
In summary, ZEST-NERF offers a significant improvement over traditional NeRF methods by reducing the computational cost and input view requirements while maintaining photo-realistic results. This makes it an attractive choice for applications where efficiency and flexibility are key, such as virtual reality and real-time rendering of dynamic scenes.
Computer Science, Computer Vision and Pattern Recognition
Scene-Agnostic Zero-Shot Novel View Synthesis with Dynamic Encoding Volume
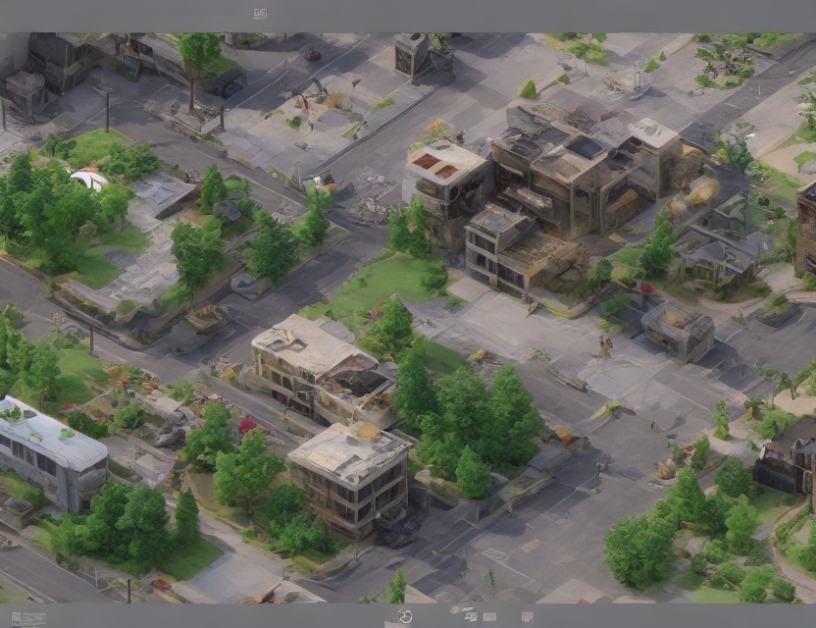