Polyps are abnormal growths on the colon wall that can increase the risk of colorectal cancer. Accurate segmentation of polyps is crucial for colonoscopy, but it’s a challenging task due to their varying sizes and shapes. To address this challenge, researchers proposed HarDNet-DFUS, a novel network that leverages attention mechanisms to focus on the most important features.
The proposed network consists of several stages, including a pyramid-structured encoder and a decoder. The encoder extracts multi-scale features from the input image using a lightweight attention mechanism, while the decoder refines the segmentation results using a combination of convolutional layers and skip connections.
To improve the segmentation performance, HarDNet-DFUS employs an adaptive scale context module that incorporates an interactive mechanism to select the most relevant features. The module uses a fuzzy attention mechanism to focus on the contextual information and combines it with the feature maps from different layers. This allows the network to capture both local and global features of the polyps, leading to more accurate segmentation results.
Another important aspect of HarDNet-DFUS is its ability to handle large-scale changes in the polyp area using an adaptive scale context module. This module is designed to address the challenge of polyps having different sizes and shapes in different areas of the colon, which can affect the accuracy of segmentation. By adapting to these changes, HarDNet-DFUS can provide more reliable segmentation results.
The proposed network also incorporates a real-time model that trades accuracy for speed, making it suitable for clinical applications. The authors evaluate the performance of HarDNet-DFUS using several metrics and show that it outperforms existing methods in terms of segmentation accuracy.
In summary, HarDNet-DFUS is a context-aware network that leverages attention mechanisms to improve the segmentation of polyps in colonoscopy images. By incorporating an adaptive scale context module and a real-time model, the proposed network can provide more accurate and efficient segmentation results for clinical applications.
Computer Science, Computer Vision and Pattern Recognition
“Selective Feature Aggregation Network for Polyp Segmentation
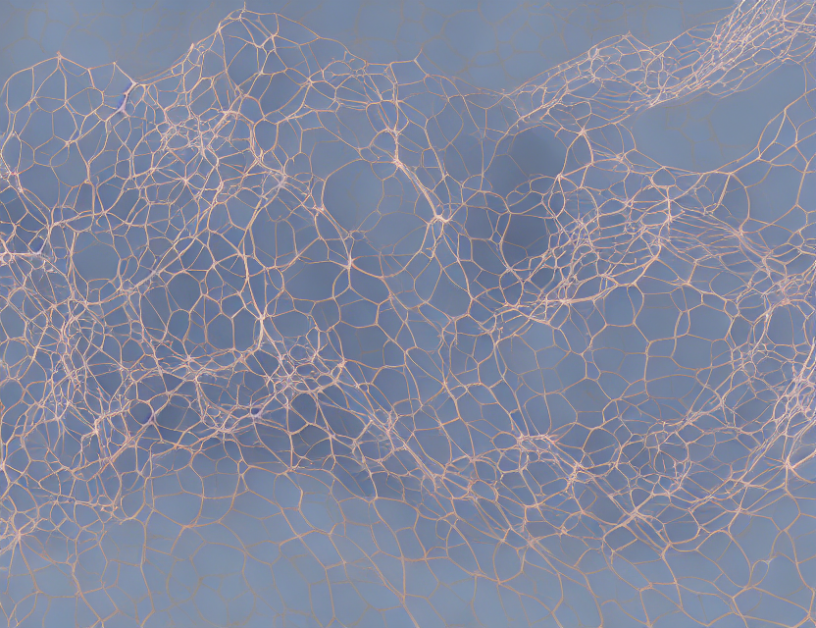