In this study, researchers explored the potential of using text-based labels to improve the performance of a general image classifier. They developed a novel method called TLDR (Text-based Labels for Debiasing) that leverages textual information to adjust the proportions of minority examination samples in the training dataset. By doing so, the model can better recognize images with less frequent labels, leading to improved overall performance.
The study demonstrated the effectiveness of TLDR by conducting an ablation study on three datasets: Waterbirds, Celebrities, and Animals. The results showed that TLDR significantly outperformed two state-of-the-art methods, AFR (Autoregressive Framework) and SELF (Semantic-based Label Embedding Framework), in recognizing images with less frequent labels. Moreover, TLDR did not require any additional training of the entire model or a class-balanced image dataset, making it a practical and efficient approach.
The researchers also explored the post-hoc utilization of baselines, which involves leveraging an already trained ERM (Expectation-Maximization) model to improve the performance of AFR and SELF in situations where additional ERM training is not feasible due to computational costs. The results showed that TLDR can be applied to pre-trained models without any additional training, which is a significant practical benefit.
In summary, this study introduced TLDR, a novel method for improving the performance of general image classifiers by leveraging textual information to adjust the proportions of minority examination samples in the training dataset. The results demonstrated the effectiveness of TLDR and its practical advantages over existing methods.
Computer Science, Computer Vision and Pattern Recognition
Semantic Filtering for Improved Text Generation
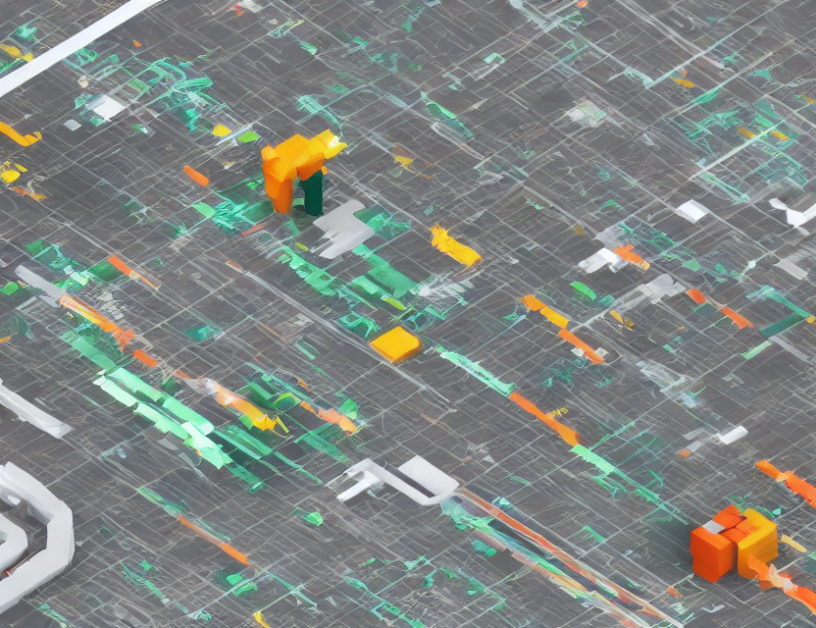