In the field of natural language processing, researchers have been working on developing methods to identify and mitigate gender bias in language models. To do this, they need a comprehensive understanding of how these models perform and what factors contribute to their biases. In their recent study, Delobelle et al. (2022) aimed to provide a more detailed picture of the state of the field by analyzing various types of language models and identifying similar patterns of behavior.
The authors used two machine learning techniques, translation and syntactic parsing, to process texts in their experiments. While these tools are useful for evaluating biases in language models, they also have limitations, such as limited accuracy and potential noise in the evaluation pipelines. By closely examining the results, the researchers found that different types of models exhibit similar patterns of behavior, indicating that they may be learning from similar sources with gender bias.
To address this issue, the authors suggest focusing on specific issues, such as how to stop models from sexualizing women. They also highlight the importance of improving the accuracy of evaluation tools and developing more fine-grained views of model behavior to address gender bias.
In conclusion, Delobelle et al. (2022) provide valuable insights into the state of the field in measuring fairness in language models. By shedding light on the limitations of current methods and identifying areas for improvement, their study paves the way for more effective approaches to addressing gender bias in these models.
Computation and Language, Computer Science
Uncovering Hidden Bias in Language Models: A Case Study
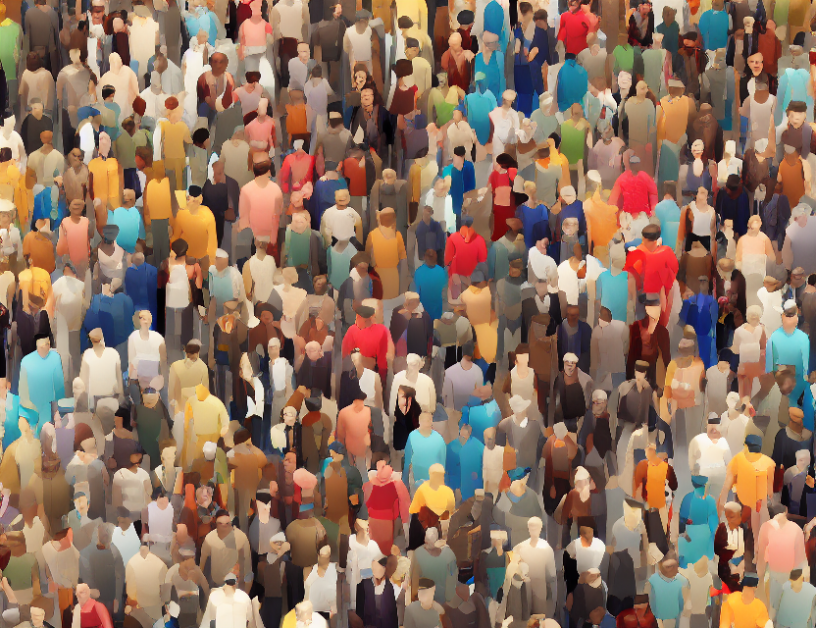