In this paper, the authors propose a novel approach to talking head synthesis called Ad-nerf, which leverages audio-driven neural radiance fields (NRF) to generate highly realistic and diverse facial animations. The Ad-nerf model is trained on a large dataset of videos with synchronized audio, allowing it to learn the relationship between audio signals and corresponding facial expressions.
The key innovation of Ad-nerf lies in its use of NRF, which enables the generation of high-quality 3D avatars that are capable of realistic speech animation. Unlike traditional talking head synthesis methods that rely on pre-defined templates or limited facial movements, Ad-nerf can generate a wide range of expressions and poses by simply providing an audio input.
To train the Ad-nerf model, the authors use a combination of unsupervised and supervised learning techniques. They first extract features from the audio data using a convolutional neural network (CNN), and then use these features to drive the NRF-based avatar animation. The model is trained on a large dataset of videos with synchronized audio, which allows it to learn the relationship between audio signals and corresponding facial expressions.
One of the main advantages of Ad-nerf is its ability to generate highly diverse and realistic facial animations. By using a probabilistic approach based on Gumbel-softmax, the model can sample from a large distribution of possible expressions and poses, resulting in more natural-looking animations than traditional methods.
The authors evaluate the performance of Ad-nerf through several experiments, demonstrating its ability to generate high-quality avatars that are consistent with the input audio. They also show that their model outperforms state-of-the-art talking head synthesis methods in terms of both subjective quality and objective metrics such as the Maximal Lip Vertex Error (MLVE).
Overall, Ad-nerf represents a significant advancement in the field of talking head synthesis, providing a powerful tool for generating highly realistic and diverse facial animations based solely on audio input. Its potential applications range from virtual reality and video games to film and television production, making it an exciting development for both researchers and practitioners in the field.
Computer Science, Computer Vision and Pattern Recognition
Unlocking Speech-Driven Animation with Diverse Datasets and Probabilistic Models
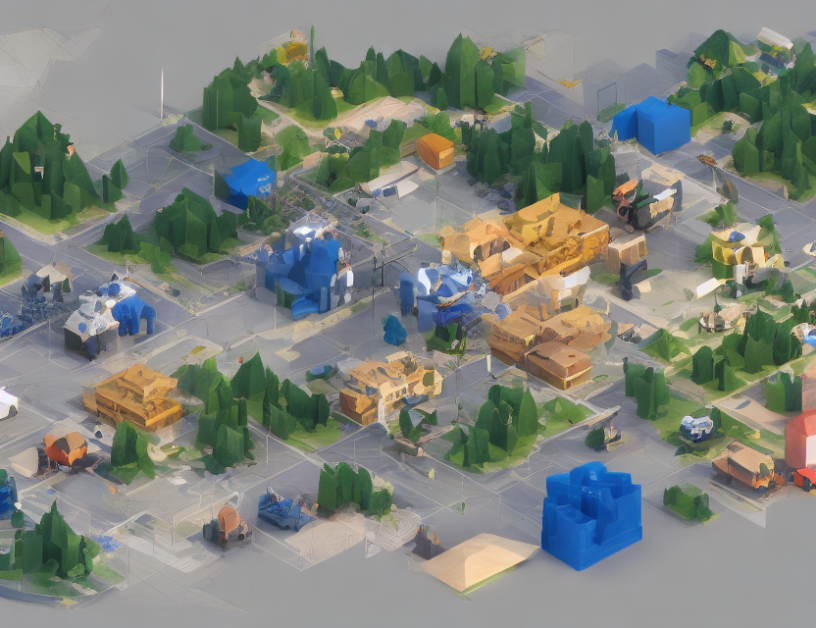